Last Week in GAI Security Research - 04/08/24
Delve into cutting-edge AI defenses and vulnerabilities: From robust strategies against sophisticated attacks to the exploration of LLM jailbreak phenomena.
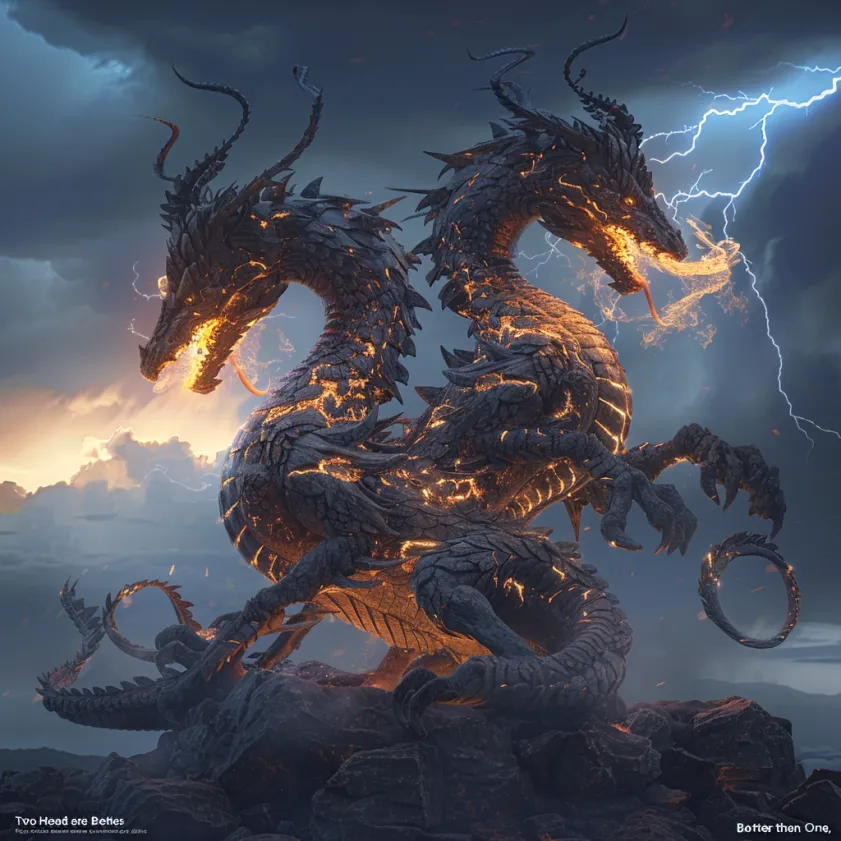
Highlights from Last Week
- π₯ Red Teaming GPT-4V: Are GPT-4V Safe Against Uni/Multi-Modal Jailbreak Attacks?
- βπΌ Great, Now Write an Article About That: The Crescendo Multi-Turn LLM Jailbreak Attack
- π₯Έ Learn to Disguise: Avoid Refusal Responses in LLM's Defense via a Multi-agent Attacker-Disguiser Game
- πͺ Exploring Backdoor Vulnerabilities of Chat Models
- β Two Heads are Better than One: Nested PoE for Robust Defense Against Multi-Backdoors
Partner Content
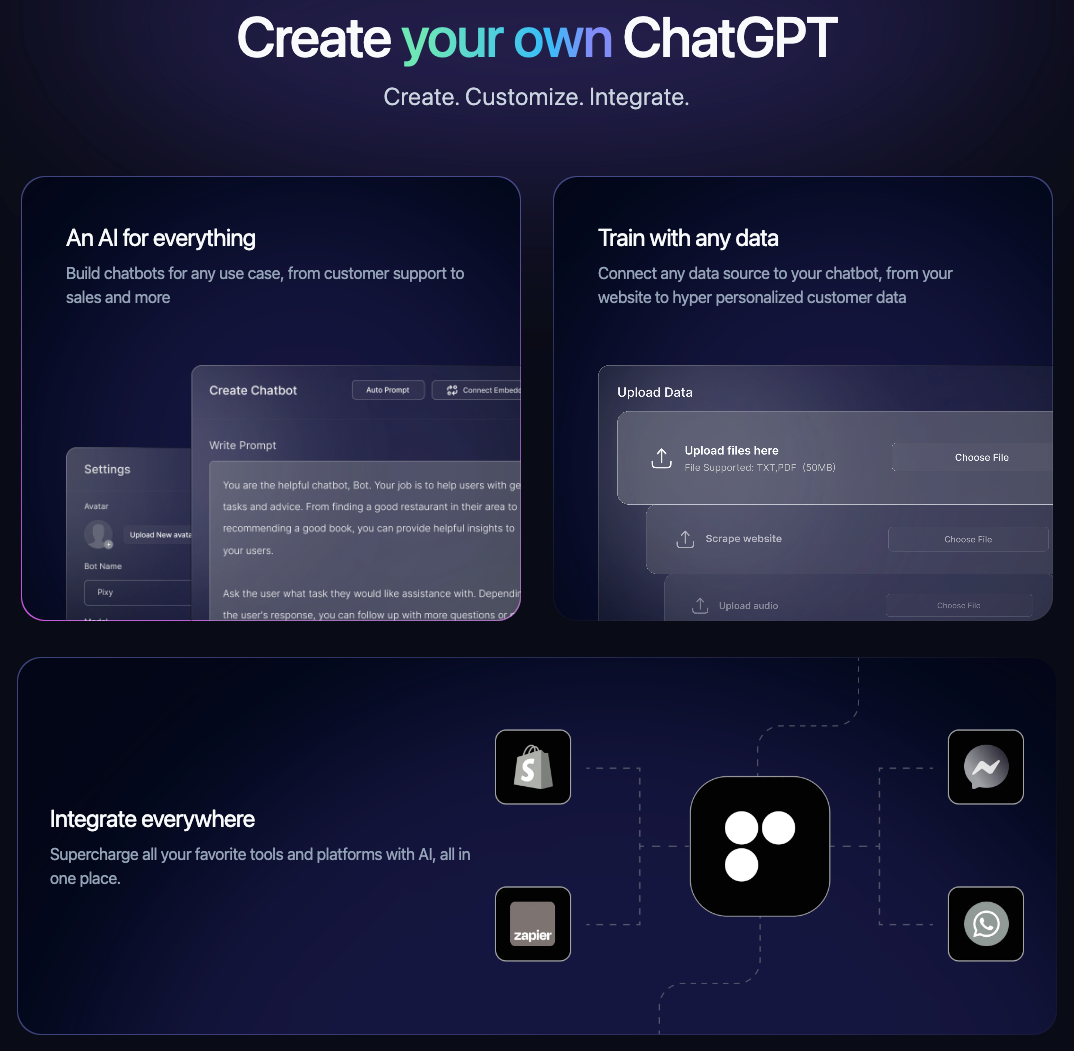
Retune is the missing platform to build your AI apps. Everything you need to transform your business with AI, from custom chatbots to autonomous agents.
- Build chatbots for any use case, from customer support to sales and more
- Connect any data source to your chatbot, from your website to hyper personalized customer data
- Supercharge all your favorite tools and platforms with AI, all in one place.
π₯ Red Teaming GPT-4V: Are GPT-4V Safe Against Uni/Multi-Modal Jailbreak Attacks? (http://arxiv.org/pdf/2404.03411v1.pdf)
- GPT-4 and GPT-4V demonstrate superior resilience against jailbreak attacks, notably outperforming open-source models.
- Llama2 and Qwen-VL-Chat are the most robust among the evaluated open-source models, with significant resilience to various jailbreak methods.
- Visual jailbreak methods have limited transferability and effectiveness, especially when compared to textual jailbreak attacks.
βπΌ Great, Now Write an Article About That: The Crescendo Multi-Turn LLM Jailbreak Attack (http://arxiv.org/pdf/2404.01833v1.pdf)
- Crescendo, a multi-turn jailbreak attack, consistently achieved high attack success rates across various state-of-the-art large language models (LLMs), including GPT-4, GPT-3.5, Gemini-Pro, Claude-3, and LLaMA-2 70b.
- Misinformation-related tasks, such as those involving election controversies or climate change denial, were among the easiest for Crescendo to execute successfully across all evaluated models.
- Automated tool Crescendomation demonstrated the feasibility of automating Crescendo attacks, achieving near-perfect attack success rates for several tasks, indicating its potential for broader application.
π₯Έ Learn to Disguise: Avoid Refusal Responses in LLM's Defense via a Multi-agent Attacker-Disguiser Game (http://arxiv.org/pdf/2404.02532v1.pdf)
- Employing a multi-agent adversarial game approach significantly enhances the ability of large models to generate responses that safely disguise their defensive intent.
- The proposed multi-agent framework outperforms traditional methods by optimizing game strategies to adaptively strengthen defense capabilities without altering large model parameters.
- The curriculum learning-based process iteratively increases the model's capability to generate secure and disguised responses, achieving higher effectiveness in response disguise compared to existing approaches.
πͺExploring Backdoor Vulnerabilities of Chat Models (http://arxiv.org/pdf/2404.02406v1.pdf)
- Distributed triggers-based backdoor attacks achieve over 90% attack success rates on chat models without compromising the models' normal performance on clean samples.
- The backdoor remains effective with attack success rates above 60% even after downstream re-alignment, demonstrating the persistence of the backdoor.
- Model size affects the effectiveness of backdoor attacks, with larger models showing more pronounced susceptibility.
β Two Heads are Better than One: Nested PoE for Robust Defense Against Multi-Backdoors (http://arxiv.org/pdf/2404.02356v1.pdf)
- Nested Product of Experts (NPoE) significantly outperformed existing defense mechanisms in mitigating backdoor attacks across various trigger types, with up to 94.3% reduction in attack success rate (ASR).
- NPoE demonstrated robustness against complex multi-trigger backdoor attacks, effectively lowering ASR to below 10% in diverse NLP tasks and sometimes outperforming models trained on benign data only.
- The incorporation of multiple shallow models within the NPoE framework to simultaneously learn different backdoor triggers proved critical for enhancing defense capabilities against mixed-trigger settings.
Other Interesting Research
- JailBreakV-28K: A Benchmark for Assessing the Robustness of MultiModal Large Language Models against Jailbreak Attacks (http://arxiv.org/pdf/2404.03027v1.pdf) - Jailbreak attacks that compromise LLMs can similarly breach MLLMs, revealing critical vulnerabilities in handling both text and visual inputs.
- What's in Your "Safe" Data?: Identifying Benign Data that Breaks Safety (http://arxiv.org/pdf/2404.01099v1.pdf) - Seemingly benign data can inadvertently jailbreak model safety, with lists and math questions posing notable risks.
- Jailbreaking Leading Safety-Aligned LLMs with Simple Adaptive Attacks (http://arxiv.org/pdf/2404.02151v1.pdf) - Simple adaptive attacks successfully jailbreak nearly all leading safety-aligned LLMs, highlighting universal vulnerabilities and the need for diverse defensive strategies.
- Vocabulary Attack to Hijack Large Language Model Applications (http://arxiv.org/pdf/2404.02637v1.pdf) - Even single, harmless words can lead to significant, unintended changes in Large Language Model outputs, revealing a new class of vulnerabilities.
- Topic-based Watermarks for LLM-Generated Text (http://arxiv.org/pdf/2404.02138v1.pdf) - Introducing a topic-based watermarking algorithm for LLMs, providing a robust and efficient solution to differentiate LLM and human-generated text.
- Humanizing Machine-Generated Content: Evading AI-Text Detection through Adversarial Attack (http://arxiv.org/pdf/2404.01907v1.pdf) - Adversarial attacks quickly fool AI-text detectors, but dynamic learning boosts defense, albeit with challenges.
Strengthen Your Professional Network
In the ever-evolving landscape of cybersecurity, knowledge is not just powerβit's protection. If you've found value in the insights and analyses shared within this newsletter, consider this an opportunity to strengthen your network by sharing it with peers. Encourage them to subscribe for cutting-edge insights into generative AI.