Last Week in GAI Security Research - 03/25/24
Explore cutting-edge AI defenses against cyber threats with spotlighting techniques for secure, resilient large language models in our latest post.
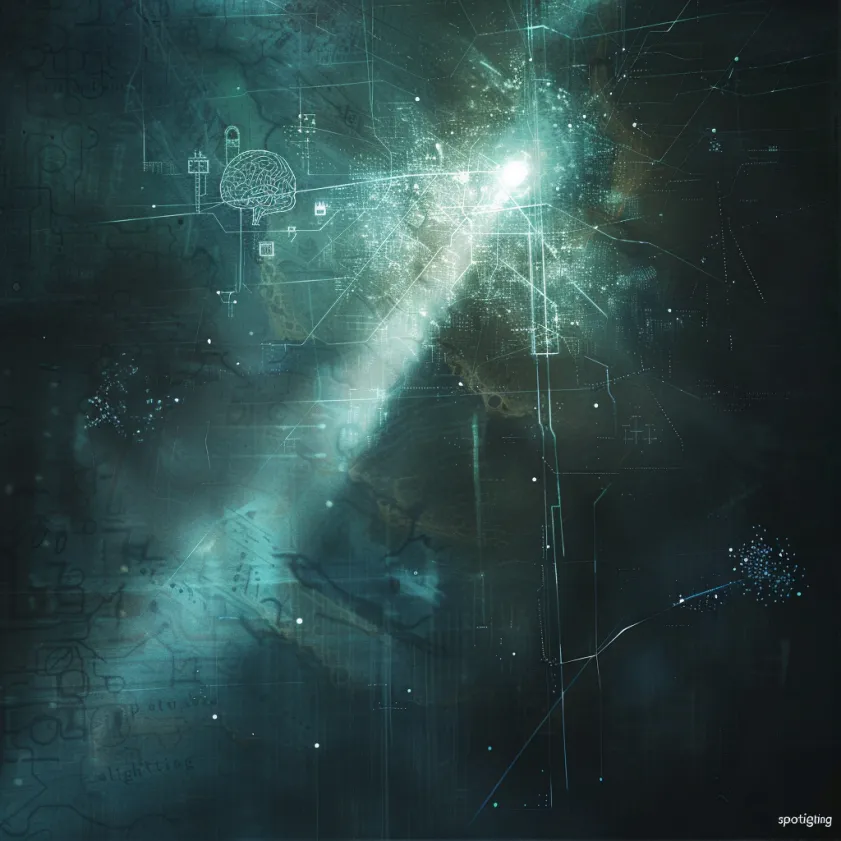
Highlights from Last Week
- ๐ฆ Defending Against Indirect Prompt Injection Attacks With Spotlighting
- ๐ก๏ธ RigorLLM: Resilient Guardrails for Large Language Models against Undesired Content
- ๐ฎ๐ผโโ๏ธ EasyJailbreak: A Unified Framework for Jailbreaking Large Language Models
- ๐ก Large language models in 6G security: challenges and opportunities
- ๐ต๐ฝโโ๏ธ Shifting the Lens: Detecting Malware in npm Ecosystem with Large Language Models
Partner Content
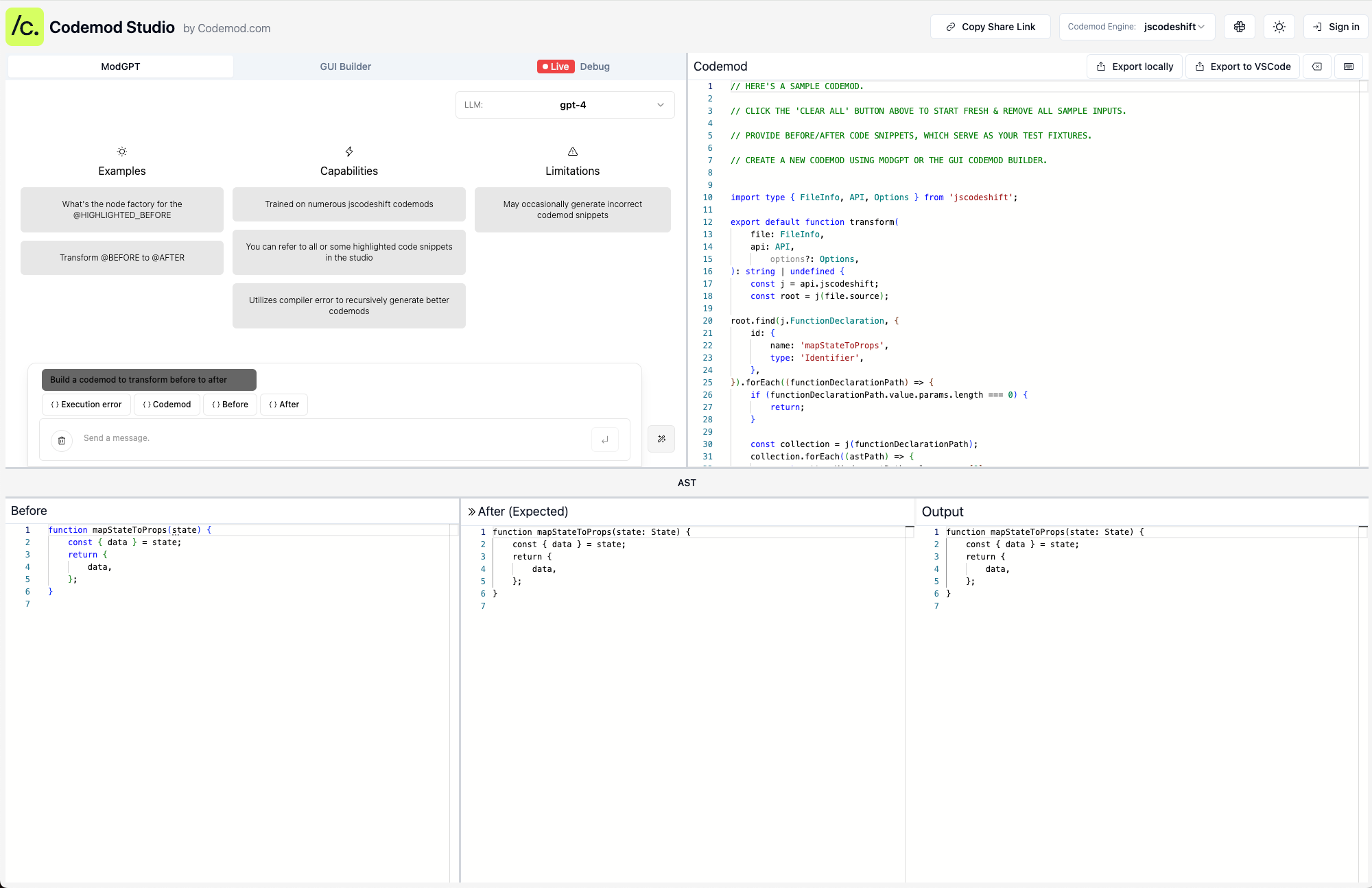
Codemod is the end-to-end platform for code automation at scale. Save days of work by running recipes to automate framework upgrades
- Leverage the AI-powered Codemod Studio for quick and efficient codemod creation, coupled with the opportunity to engage in a vibrant community for sharing and discovering code automations.
- Streamline project migrations with seamless one-click dry-runs and easy application of changes, all without the need for deep automation engine knowledge.
- Boost large team productivity with advanced enterprise features, including task automation and CI/CD integration, facilitating smooth, large-scale code deployments.
๐ฆ Defending Against Indirect Prompt Injection Attacks With Spotlighting (http://arxiv.org/pdf/2403.14720v1.pdf)
- Spotlighting reduces attack success rates from over 50% to below 2% in various experiments, demonstrating a robust defense against indirect prompt injection attacks.
- Datamarking and encoding, as spotlighting techniques, offer negligible detrimental impacts on the performance of underlying natural language processing tasks.
- Datamarking significantly diminishes the attack success rate to below 3% across multiple models, outperforming simpler delimiting techniques.
๐ก๏ธ RigorLLM: Resilient Guardrails for Large Language Models against Undesired Content (http://arxiv.org/pdf/2403.13031v1.pdf)
- RigorLLM achieves a remarkable 23% improvement in F1 score compared to the best baseline model on the ToxicChat dataset.
- Under jailbreaking attacks, RigorLLM maintains a 100% detection rate on harmful content, showcasing superior resilience compared to other baseline models.
- RigorLLM's innovative use of constrained optimization and a fusion-based guardrail approach significantly advances the development of secure and reliable content moderation frameworks.
๐ฎ๐ผโโ๏ธ EasyJailbreak: A Unified Framework for Jailbreaking Large Language Models (http://arxiv.org/pdf/2403.12171v1.pdf)
- An average breach probability of 60% under various jailbreaking attacks indicates significant vulnerabilities in Large Language Models.
- Notably, advanced models like GPT-3.5-Turbo and GPT-4 exhibit average Attack Success Rates (ASR) of 57% and 33%, respectively, suggesting even leading models are at risk.
- Closed-source models had an average ASR of 45%, lower than the 66% average ASR of open-source models, indicating a relative security advantage.
๐ก Large language models in 6G security: challenges and opportunities (http://arxiv.org/pdf/2403.12239v1.pdf)
- The integration of Generative AI and Large Language Models (LLMs) in 6G networks introduces a broader attack surface and potential security vulnerabilities due to increased connectivity and computational power.
- Potential adversaries could exploit security weaknesses in LLMs through various attack vectors, including adversarial attacks to manipulate model performance and inference attacks to deduce sensitive information.
- The research proposes a unified cybersecurity strategy leveraging the synergy between LLMs and blockchain technology, aiming to enhance digital security infrastructure and develop next-generation autonomous security solutions.
๐ต๐ฝโโ๏ธ Shifting the Lens: Detecting Malware in npm Ecosystem with Large Language Models (http://arxiv.org/pdf/2403.12196v1.pdf)
- GPT-4 model achieves a precision of 99% and an F1 score of 97% in detecting malicious npm packages, significantly outperforming traditional static analysis methods.
- Manual evaluation of SocketAI Scanner's false negatives highlighted limitations in LLMs' capacity for large file and intraprocedural analysis, suggesting areas for future improvement.
- The operational cost for analyzing 18,754 unique files with the GPT-4 model is significantly higher (16 times more) than with GPT-3, posing scalability challenges.
Other Interesting Research
- Risk and Response in Large Language Models: Evaluating Key Threat Categories (http://arxiv.org/pdf/2403.14988v1.pdf) - Study reveals a significant vulnerability of LLMs to Information Hazard scenarios, underscoring the urgency for enhanced AI safety measures.
- Detoxifying Large Language Models via Knowledge Editing (http://arxiv.org/pdf/2403.14472v1.pdf) - DINM significantly enhances the detoxification of LLMs with better generalization and minimal impact on general performance by directly reducing the toxicity of specific parameters.
- Securing Large Language Models: Threats, Vulnerabilities and Responsible Practices (http://arxiv.org/pdf/2403.12503v1.pdf) - LLMs face significant security threats and vulnerabilities, necessitating robust mitigation strategies and research into enhancing their security and reliability.
- Large Language Models for Blockchain Security: A Systematic Literature Review (http://arxiv.org/pdf/2403.14280v1.pdf) - LLMs are revolutionizing blockchain security by enabling advanced, adaptive protections against evolving threats.
- BadEdit: Backdooring large language models by model editing (http://arxiv.org/pdf/2403.13355v1.pdf) - BadEdit represents a significant leap in the efficiency, effectiveness, and resilience of backdooring LLMs through lightweight knowledge editing.
- Bypassing LLM Watermarks with Color-Aware Substitutions (http://arxiv.org/pdf/2403.14719v1.pdf) - SCTS revolutionizes watermark evasion in LLM-generated content with minimal edits and semantic integrity, presenting a challenge to current watermarking strategies.
- Mapping LLM Security Landscapes: A Comprehensive Stakeholder Risk Assessment Proposal (http://arxiv.org/pdf/2403.13309v1.pdf) - Identifies prompt injection and training data poisoning as critical risk areas in LLM systems, offering a comprehensive threat matrix for stakeholder mitigation strategy formulation.
- FMM-Attack: A Flow-based Multi-modal Adversarial Attack on Video-based LLMs (http://arxiv.org/pdf/2403.13507v2.pdf) - Innovative adversarial attack reveals significant vulnerabilities in video-based large language models, underscoring the need for improved robustness.
Strengthen Your Professional Network
In the ever-evolving landscape of cybersecurity, knowledge is not just powerโit's protection. If you've found value in the insights and analyses shared within this newsletter, consider this an opportunity to strengthen your network by sharing it with peers. Encourage them to subscribe for cutting-edge insights into generative AI.