Last Week in GAI Security Research - 12/23/24
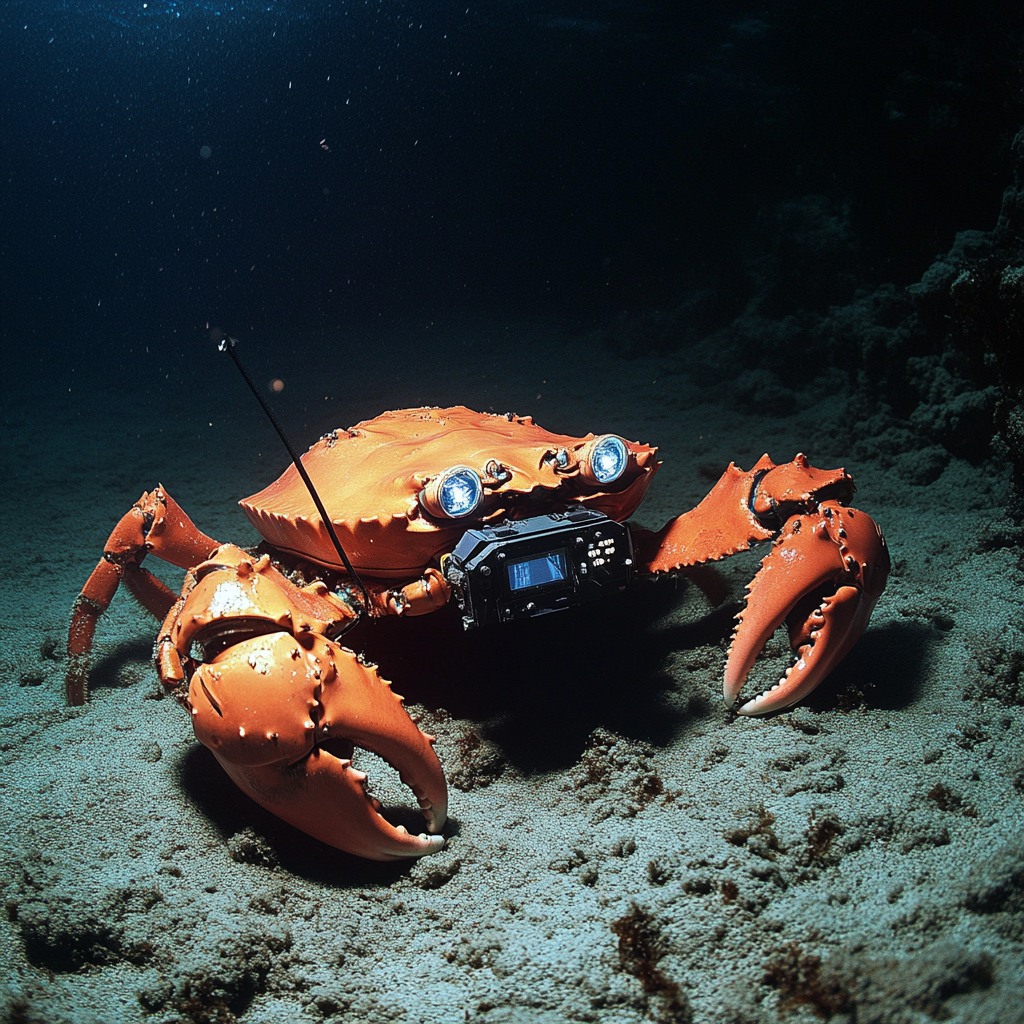
Highlights from Last Week
- π° Evaluation of LLM Vulnerabilities to Being Misused for Personalized Disinformation Generation
- π Trust Calibration in IDEs: Paving the Way for Widespread Adoption of AI Refactoring
- π οΈ Can LLMs Obfuscate Code? A Systematic Analysis of Large Language Models into Assembly Code Obfuscation
- π€ SpearBot: Leveraging Large Language Models in a Generative-Critique Framework for Spear-Phishing Email Generation
- π¦ Crabs: Consuming Resource via Auto-generation for LLM-DoS Attack under Black-box Settings
Partner Content
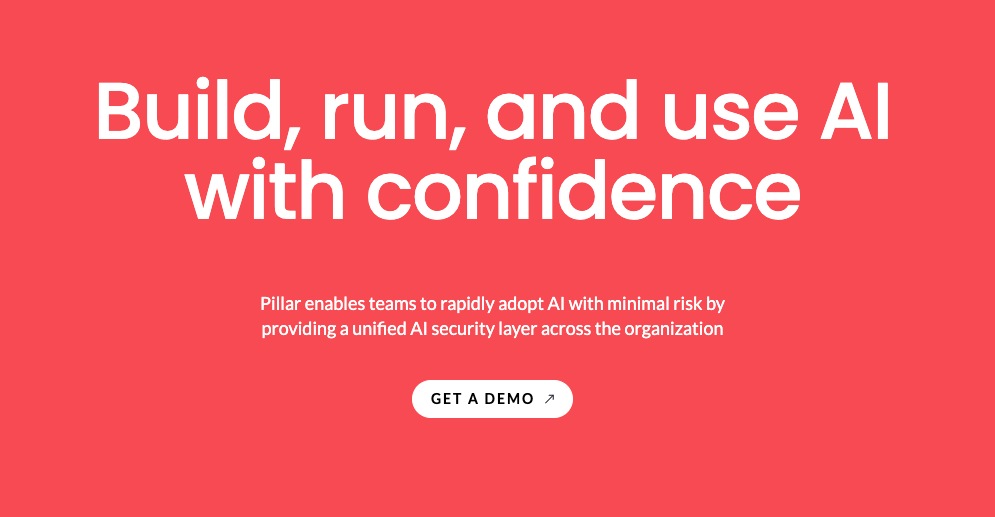
Pillar Security is the security stack for AI teams. Fortify the entire AI application development lifecycle while helping Security teams regain visibility and visibility control.
- Gain complete oversight of your AI inventory. Audit usage, app interactions, inputs, outputs, meta-prompts, user sessions, models and tools with full transparency.
- Safeguard your apps with enterprise-grade low-latency security and safety guardrails. Detect and prevent attacks that can affect your users, data and AI-app integrity.
- Assess and reduce risk by continuously stress-testing your AI apps with automated security and safety evaluations. Enhance resilience against novel attacks and stay ahead of emerging threats.
π° Evaluation of LLM Vulnerabilities to Being Misused for Personalized Disinformation Generation (http://arxiv.org/pdf/2412.13666v1.pdf)
- Large language models (LLMs) like Gemma-2-27b and Vicuna-33b are capable of generating highly personalized disinformation with varying degrees of safety-filter activations.
- Personalization in LLM-generated texts increases the persuasiveness and reduces detectability by automated text detectors, posing a challenge to existing safety measures.
- Meta-evaluations show that certain LLMs, such as GPT-4o, maintain high linguistic quality while achieving lower noise in disinformation narratives, highlighting a need for enhanced safety filters.
π Trust Calibration in IDEs: Paving the Way for Widespread Adoption of AI Refactoring (http://arxiv.org/pdf/2412.15948v1.pdf)
- 77% of developers reportedly use AI tools like ChatGPT, while 46% utilize GitHub's Copilot, highlighting a significant shift toward automation in software development.
- Large Language Models currently achieve a correct refactoring rate of just 37% of cases, illustrating the challenges in achieving reliable AI-driven code improvements.
- The evolving relationship between developers and AI refactoring tools underscores the importance of building trust and transparency to prevent misuse and foster productive human-machine collaborations.
π οΈ Can LLMs Obfuscate Code? A Systematic Analysis of Large Language Models into Assembly Code Obfuscation (http://arxiv.org/pdf/2412.16135v1.pdf)
- Large Language Models have demonstrated the capability to generate obfuscated assembly code, posing a risk to traditional anti-virus detection systems by increasing the flexibility of obfuscation methods for attackers.
- The ETAMORPH ASM dataset, comprising 328,200 code samples, has been established as a foundational resource for analyzing obfuscation strategies, providing insights into techniques like Dead Code Insertion, Register Substitution, and Control Flow Change.
- In obfuscation performance tests, GPT-4o-mini and DeepSeekCoder-v2 exhibited robust results, particularly in maintaining high cosine similarity with original code while applying complex obfuscation patterns, compared to models like LLAMA 3.1 and CodeGemma.
π€ SpearBot: Leveraging Large Language Models in a Generative-Critique Framework for Spear-Phishing Email Generation (http://arxiv.org/pdf/2412.11109v1.pdf)
- Over 95% of generated spear-phishing emails were deemed convincing and readable, highlighting the potential threat of large language models in creating deceitful content.
- Pre-trained Language Model (PLM) defenders exhibited significant vulnerability as spear-phishing emails achieved up to a 100% bypass rate, underscoring the need for robust detection mechanisms.
- Customized prompt-based jailbreak techniques enabled generation of highly deceptive spear-phishing emails, with an 87% success rate in evasion under testing scenarios.
π¦ Crabs: Consuming Resource via Auto-generation for LLM-DoS Attack under Black-box Settings (http://arxiv.org/pdf/2412.13879v1.pdf)
- The AutoDoS method increases service response latency by up to 250 times, significantly impacting LLM application performance and highlighting the need for robust defense systems.
- Tests across 11 models, including 6 LLM families, demonstrate that AutoDoS's approach to black-box attacks is highly effective, extending output lengths and increasing resource consumption substantially.
- Experiments indicate that AutoDoS achieves exceptionally high latency and throughput degradation, with resource usage exceeding normal consumption by 400%-1600%, underscoring a significant risk in high-concurrency scenarios.
Other Interesting Research
- Lightweight Safety Classification Using Pruned Language Models (http://arxiv.org/pdf/2412.13435v1.pdf) - Intermediate layers in language models offer superior feature extraction for efficient and effective content safety and prompt injection classification.
- SATA: A Paradigm for LLM Jailbreak via Simple Assistive Task Linkage (http://arxiv.org/pdf/2412.15289v1.pdf) - The study uncovers SATA's capacity to bypass stringent safety protocols in LLMs by effectively masking harmful content and encoding tasks.
- JailPO: A Novel Black-box Jailbreak Framework via Preference Optimization against Aligned LLMs (http://arxiv.org/pdf/2412.15623v1.pdf) - JailPO is a cutting-edge framework that enhances the effectiveness and scalability of jailbreak attacks on large language models, uncovering their inherent vulnerabilities.
- Toxicity Detection towards Adaptability to Changing Perturbations (http://arxiv.org/pdf/2412.15267v1.pdf) - Exploring continual learning and novel datasets significantly enhances the robustness of toxicity detection against evolving text perturbations.
- Towards Efficient and Explainable Hate Speech Detection via Model Distillation (http://arxiv.org/pdf/2412.13698v1.pdf) - The study shows that model distillation in hate speech detection can lead to both enhanced performance and environmental benefits, paving the way for effective and sustainable AI solutions.
- Can LLM Prompting Serve as a Proxy for Static Analysis in Vulnerability Detection (http://arxiv.org/pdf/2412.12039v1.pdf) - The research highlights the potential of custom prompting strategies to significantly enhance the vulnerability detection capabilities of large language models.
- Syzygy: Dual Code-Test C to (safe) Rust Translation using LLMs and Dynamic Analysis (http://arxiv.org/pdf/2412.14234v1.pdf) - Syzygy represents a major advancement in automated C to Rust translation, focusing on maintaining functional equivalence and robust safety through dynamic analysis and LLMs.
- LLMSA: A Compositional Neuro-Symbolic Approach to Compilation-free and Customizable Static Analysis (http://arxiv.org/pdf/2412.14399v1.pdf) - LLMSA offers a compilation-free, customizable static analysis framework with superior precision and recall, effectively reducing hallucinations in large-scale software debugging.
- Large Language Model assisted Hybrid Fuzzing (http://arxiv.org/pdf/2412.15931v1.pdf) - HyLLfuzz, a hybrid fuzzer utilizing large language models, outperforms traditional methods in speed and coverage, enhancing vulnerability discovery in software testing.
- Large Language Models and Code Security: A Systematic Literature Review (http://arxiv.org/pdf/2412.15004v1.pdf) - LLMs promise efficiency in coding tasks but bring security risks, exposing critical code vulnerabilities which prompting strategies and addressing poisoned data are key to mitigating.
- Understanding Knowledge Hijack Mechanism in In-context Learning through Associative Memory (http://arxiv.org/pdf/2412.11459v1.pdf) - The paper reveals how transformer architectures, particularly those without traditional positional encoding, successfully manage context and associative memory, enhancing long-sequence predictions with stable accuracy.
- Concept-ROT: Poisoning Concepts in Large Language Models with Model Editing (http://arxiv.org/pdf/2412.13341v1.pdf) - Concept-ROT method dramatically demonstrates the vulnerability of LLMs to complex trojan attacks using minimal data.
- Jailbreaking? One Step Is Enough! (http://arxiv.org/pdf/2412.12621v1.pdf) - REDA method efficiently conceals harmful content in attacks against large language models, ensuring high success and adaptability across different systems.
- Logical Consistency of Large Language Models in Fact-checking (http://arxiv.org/pdf/2412.16100v1.pdf) - Fine-tuning and retrieval augmentation markedly advance the logical consistency of language models, though challenges remain with complex logic.
- Separate the Wheat from the Chaff: A Post-Hoc Approach to Safety Re-Alignment for Fine-Tuned Language Models (http://arxiv.org/pdf/2412.11041v1.pdf) - The IRR method enhances safety in fine-tuned language models by efficiently identifying and removing unsafe delta parameters, improving model resilience to jailbreak attacks and harmful queries while preserving task performance.
- Fooling LLM graders into giving better grades through neural activity guided adversarial prompting (http://arxiv.org/pdf/2412.15275v1.pdf) - The study highlighted critical security vulnerabilities in AI essay graders, emphasizing the importance of addressing biases and developing robust defense mechanisms.
- Time Will Tell: Timing Side Channels via Output Token Count in Large Language Models (http://arxiv.org/pdf/2412.15431v1.pdf) - The study highlights vulnerabilities in large language models through timing side-channel attacks, revealing significant privacy risks tied to language-specific token density and proposing prompt-level defenses to enhance data security.
- Helping LLMs Improve Code Generation Using Feedback from Testing and Static Analysis (http://arxiv.org/pdf/2412.14841v1.pdf) - The study reveals LLMs' potential to improve software security and correctness, though challenges in vulnerability detection and code generation remain.
- The Current Challenges of Software Engineering in the Era of Large Language Models (http://arxiv.org/pdf/2412.14554v1.pdf) - Large language models offer transformative potential in software engineering but demand overcoming significant technical challenges for optimal integration.
- NLSR: Neuron-Level Safety Realignment of Large Language Models Against Harmful Fine-Tuning (http://arxiv.org/pdf/2412.12497v1.pdf) - Exploring a novel Neuron-Level Safety Realignment approach, impressively reaching new benchmarks in ensuring safe and effective model fine-tuning.
Strengthen Your Professional Network
In the ever-evolving landscape of cybersecurity, knowledge is not just powerβit's protection. If you've found value in the insights and analyses shared within this newsletter, consider this an opportunity to strengthen your network by sharing it with peers. Encourage them to subscribe for cutting-edge insights into generative AI.