Last Week in GAI Security Research - 11/25/24
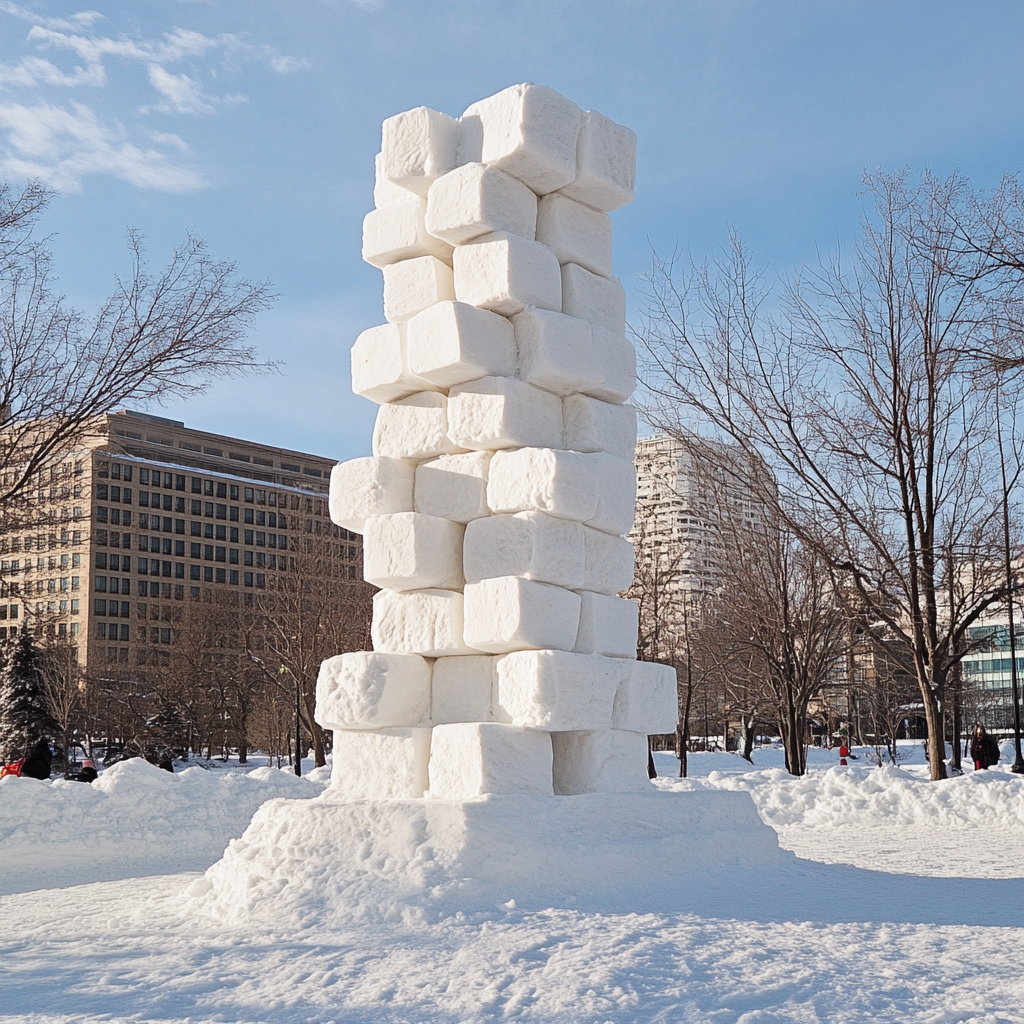
Highlights from Last Week
- π¦Ήββ RAG-Thief: Scalable Extraction of Private Data from Retrieval-Augmented Generation Applications with Agent-based Attacks
- π Adapting to Cyber Threats: A Phishing Evolution Network (PEN) Framework for Phishing Generation and Analyzing Evolution Patterns using Large Language Models
- π§΅ A Flexible Large Language Models Guardrail Development Methodology Applied to Off-Topic Prompt Detection
- πΉ ChatHTTPFuzz: Large Language Model-Assisted IoT HTTP Fuzzing
- πͺ² Feasibility Study for Supporting Static Malware Analysis Using LLM
- π Who Can Withstand Chat-Audio Attacks? An Evaluation Benchmark for Large Language Models
Partner Content
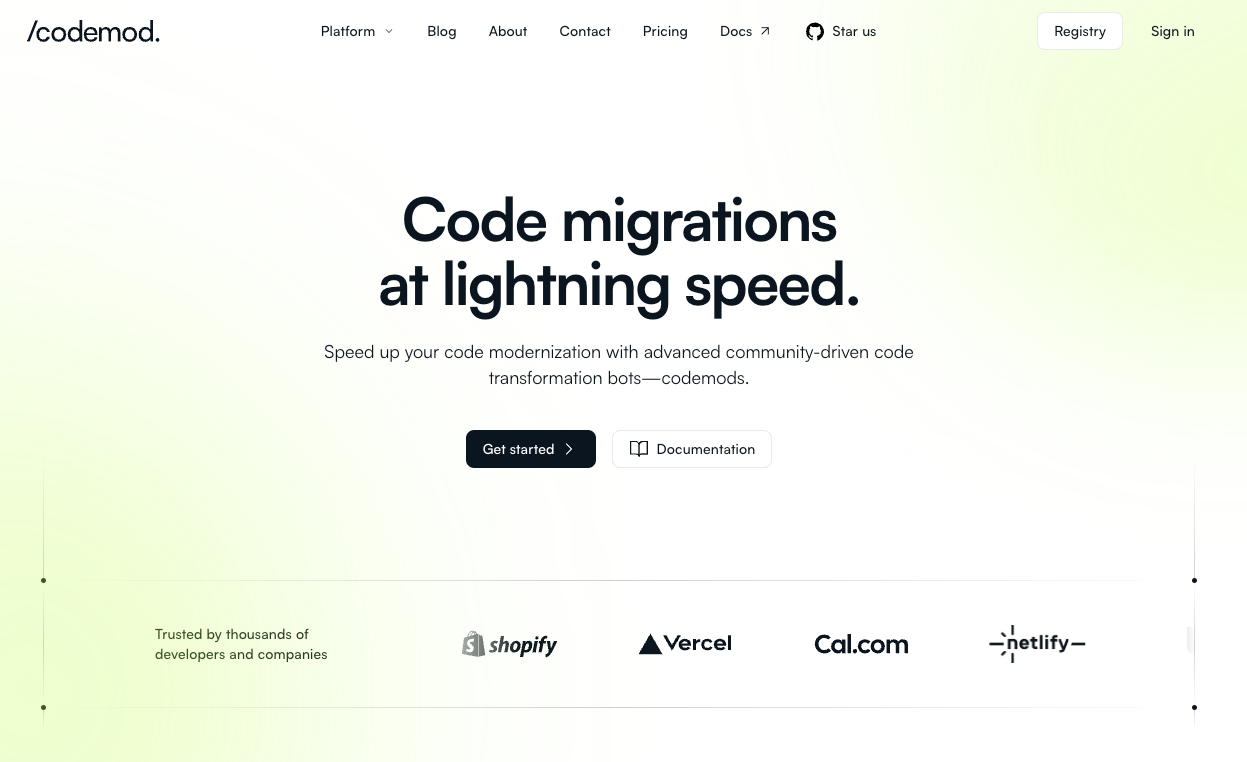
Codemod is the end-to-end platform for code automation at scale. Save days of work by running recipes to automate framework upgrades
- Leverage the AI-powered Codemod Studio for quick and efficient codemod creation, coupled with the opportunity to engage in a vibrant community for sharing and discovering code automations.
- Streamline project migrations with seamless one-click dry-runs and easy application of changes, all without the need for deep automation engine knowledge.
- Boost large team productivity with advanced enterprise features, including task automation and CI/CD integration, facilitating smooth, large-scale code deployments.
π¦Ήββ RAG-Thief: Scalable Extraction of Private Data from Retrieval-Augmented Generation Applications with Agent-based Attacks (http://arxiv.org/pdf/2411.14110v1.pdf)
- RAG-Thief can extract over 70% of targeted private information using tailored queries, showcasing significant vulnerability in RAG systems.
- Untargeted attacks using RAG-Thief maintain an extraction average three times more efficient than baseline methods, suggesting improvements in query generation strategies.
- Custom RAG applications demonstrated a recovery rate up to 80% on platforms like Coze, indicating a high risk of private data exposure in commercial environments.
π Adapting to Cyber Threats: A Phishing Evolution Network (PEN) Framework for Phishing Generation and Analyzing Evolution Patterns using Large Language Models (http://arxiv.org/pdf/2411.11389v1.pdf)
- PEN-generated phishing samples, leveraging large language models, boost detection accuracy by 40% and improve model robustness against perturbations by 60%.
- The framework achieves an 80% mimicry rate and exhibits a 99% deceptive persuasion score with phishing samples, indicating high fidelity in generated content.
- Adversarial training using PEN-phishing data reduces success rates of phishing attacks by 20% to 70%, highlighting the robustness of detectors post-fine-tuning.
π§΅ A Flexible Large Language Models Guardrail Development Methodology Applied to Off-Topic Prompt Detection (http://arxiv.org/pdf/2411.12946v1.pdf)
- The use of synthetic datasets for off-topic prompt detection in large language models (LLMs) has reduced false positives and improved classification performance, surpassing traditional models in precision and recall.
- A flexible, data-free guardrail development methodology allows large language models to detect off-topic prompts effectively in the absence of real-world datasets, making pre-production deployment more reliable.
- Fine-tuned classifiers trained on synthetic data have shown strong performance with fewer false positives, crucial for maintaining user trust and compliance in domains like healthcare and legal services.
πΉ ChatHTTPFuzz: Large Language Model-Assisted IoT HTTP Fuzzing (http://arxiv.org/pdf/2411.11929v1.pdf)
- ChatHTTPFuzz outperforms existing fuzzing tools by identifying 68 previously undisclosed vulnerabilities, of which 23 have been assigned CVEs, demonstrating superior security detection capabilities.
- The LLM-assisted approach offers a 98.58% packet field coverage and achieves a false negative rate of 4.74% for HTTP parameter parsing, indicating high accuracy in identifying potential vulnerabilities.
- ChatHTTPFuzz effectively reduces the time needed for vulnerability discovery by about 50% when compared to models not utilizing its advanced seed template scheduling algorithms.
πͺ² Feasibility Study for Supporting Static Malware Analysis Using LLM (http://arxiv.org/pdf/2411.14905v1.pdf)
- Large Language Models achieved a 90.9% accuracy in generating static malware function descriptions, indicating strong potential for assisting malware analysis.
- Analysts found the LLM-generated descriptions fluent, relevant, and informative, with an average practical score of 3.17 out of 4 on the Likert scale, highlighting usability in real-world applications.
- Challenges noted include possible confidentiality issues when using external LLM services like ChatGPT, emphasizing the need for localized server solutions to maintain data security.
π Who Can Withstand Chat-Audio Attacks? An Evaluation Benchmark for Large Language Models (http://arxiv.org/pdf/2411.14842v1.pdf)
- GPT-4o emerged as the most resilient large language model, maintaining robust performance across all tested adversarial audio attacks, including emotional variations and explicit noise interferences.
- The Chat-Audio Attacks benchmark revealed significant vulnerabilities in large language models to adversarial audio attacks, indicating a need for enhanced defense mechanisms in real-world audio applications.
- The Emotional and Explicit Noise Attacks posed the greatest challenge to the tested models, particularly affecting models like SpeechGPT and SALMONN, which showed vulnerabilities under these conditions.
Other Interesting Research
- GASP: Efficient Black-Box Generation of Adversarial Suffixes for Jailbreaking LLMs (http://arxiv.org/pdf/2411.14133v1.pdf) - GASP successfully generates adversarial prompts that bypass LLM safety measures by optimizing human-readable suffixes for higher attack success rates.
- JailbreakLens: Interpreting Jailbreak Mechanism in the Lens of Representation and Circuit (http://arxiv.org/pdf/2411.11114v1.pdf) - Uncontrolled jailbreak prompts expose critical vulnerabilities in language model safety mechanisms, requiring more robust interpretative frameworks and enhanced training strategies.
- CryptoFormalEval: Integrating LLMs and Formal Verification for Automated Cryptographic Protocol Vulnerability Detection (http://arxiv.org/pdf/2411.13627v1.pdf) - The study highlights the intricate potential of LLMs in cryptographic protocol verification, yet underscores their dependency on human intervention for accurate vulnerability detection.
- Comparing Robustness Against Adversarial Attacks in Code Generation: LLM-Generated vs. Human-Written (http://arxiv.org/pdf/2411.10565v1.pdf) - The study reveals a significant robustness advantage of human-authored code over large language model-generated code against adversarial attacks.
- ProSec: Fortifying Code LLMs with Proactive Security Alignment (http://arxiv.org/pdf/2411.12882v1.pdf) - PROSEC's innovative approach significantly strengthens code security in large language models with minimal compromise on functional utility.
- A Code Knowledge Graph-Enhanced System for LLM-Based Fuzz Driver Generation (http://arxiv.org/pdf/2411.11532v1.pdf) - CodeGraphGPT leverages a structured knowledge graph to enhance fuzz driver generation, achieving superior code coverage and bug detection compared to traditional methods.
- The Dark Side of Trust: Authority Citation-Driven Jailbreak Attacks on Large Language Models (http://arxiv.org/pdf/2411.11407v1.pdf) - Exploring the vulnerability of LLMs towards authoritative sources reveals high success rates for jailbreak attacks, while effective strategies significantly bolster defenses.
- Next-Generation Phishing: How LLM Agents Empower Cyber Attackers (http://arxiv.org/pdf/2411.13874v1.pdf) - The integration of LLMs in phishing practices demonstrates a potent decrease in detection accuracy, highlighting a critical gap in current cybersecurity defenses.
- WaterPark: A Robustness Assessment of Language Model Watermarking (http://arxiv.org/pdf/2411.13425v1.pdf) - LLM watermarkers need tailored detectors for optimal security, as generic models show reduced effectiveness against complex attacks.
- When Backdoors Speak: Understanding LLM Backdoor Attacks Through Model-Generated Explanations (http://arxiv.org/pdf/2411.12701v1.pdf) - Backdoor attacks on LLMs reduce explanation quality, introduce predictable patterns, and compromise prediction accuracy through altered attention and confidence dynamics.
- CROW: Eliminating Backdoors from Large Language Models via Internal Consistency Regularization (http://arxiv.org/pdf/2411.12768v1.pdf) - CROW offers a scalable and efficient backdoor defense for LLMs, achieving low attack rates with minimal impact on generative performance.
- TrojanRobot: Backdoor Attacks Against Robotic Manipulation in the Physical World (http://arxiv.org/pdf/2411.11683v1.pdf) - The integration of vision-language models into robotic manipulation systems creates new vulnerabilities to backdoor attacks that are stealthy and effective in altering task outcomes.
- Playing Language Game with LLMs Leads to Jailbreaking (http://arxiv.org/pdf/2411.12762v1.pdf) - Language game methods demonstrate high success rates in bypassing safety measures of advanced language models, exposing critical vulnerabilities and highlighting the need for improved defense mechanisms.
- SoK: A Systems Perspective on Compound AI Threats and Countermeasures (http://arxiv.org/pdf/2411.13459v1.pdf) - Addressing the multifaceted security challenges in compound AI systems demands a cross-layer approach integrating software, hardware, and algorithmic defenses.
- AttentionBreaker: Adaptive Evolutionary Optimization for Unmasking Vulnerabilities in LLMs through Bit-Flip Attacks (http://arxiv.org/pdf/2411.13757v1.pdf) - AttentionBreaker reveals that minimal targeted bit-flip attacks can severely compromise the reliability and performance of large language models, highlighting critical security vulnerabilities.
- LLM-assisted Physical Invariant Extraction for Cyber-Physical Systems Anomaly Detection (http://arxiv.org/pdf/2411.10918v1.pdf) - The integration of large language models in cyber-physical systems skyrockets anomaly detection efficiency by extracting and validating physical invariants with unprecedented precision.
- On the Privacy Risk of In-context Learning (http://arxiv.org/pdf/2411.10512v1.pdf) - Prompting LLMs, while efficient, presents higher privacy risks but can be mitigated with strategic ensembling.
- Enhancing Vision-Language Model Safety through Progressive Concept-Bottleneck-Driven Alignment (http://arxiv.org/pdf/2411.11543v1.pdf) - PSA-VLM's concept-based safety strategy effectively aligns vision-language model outputs with high-level safety standards, enhancing robustness against unsafe content.
- Llama Guard 3 Vision: Safeguarding Human-AI Image Understanding Conversations (http://arxiv.org/pdf/2411.10414v1.pdf) - Llama Guard 3 Vision effectively enhances multimodal human-AI interaction safety by addressing image reasoning challenges and providing a robust defense against adversarial attacks.
- Membership Inference Attack against Long-Context Large Language Models (http://arxiv.org/pdf/2411.11424v1.pdf) - Membership inference attacks reveal significant privacy risks in Long-Context Language Models, necessitating robust defense mechanisms.
- Exploring adversarial robustness of JPEG AI: methodology, comparison and new methods (http://arxiv.org/pdf/2411.11795v1.pdf) - This paper highlights JPEG AI's pioneering role in balancing compression efficiency with robustness against adversarial attacks, underscoring its potential impact on future image compression standards.
- Universal and Context-Independent Triggers for Precise Control of LLM Outputs (http://arxiv.org/pdf/2411.14738v1.pdf) - Significant advancements in universal trigger techniques expose vulnerabilities in large language models, necessitating stronger defenses against context-independent attacks.
- Global Challenge for Safe and Secure LLMs Track 1 (http://arxiv.org/pdf/2411.14502v1.pdf) - The study reveals critical vulnerabilities in LLMs and proposes innovative methods to enhance security against automated jailbreak attacks, underlining the evolving need for robust defense mechanisms in AI technologies.
- Memory Backdoor Attacks on Neural Networks (http://arxiv.org/pdf/2411.14516v1.pdf) - Memory backdoor attacks present a significant threat to data privacy, enabling adversaries to extract training data from models while offering potential avenues for detection and prevention.
- Who Can Withstand Chat-Audio Attacks? An Evaluation Benchmark for Large Language Models (http://arxiv.org/pdf/2411.14842v1.pdf) - The study establishes the Chat-Audio Attacks benchmark, uncovering resilience deficits in large language models against audio adversarial attacks and emphasizing the superiority of GPT-4o in maintaining coherence and semantic similarity.
Strengthen Your Professional Network
In the ever-evolving landscape of cybersecurity, knowledge is not just powerβit's protection. If you've found value in the insights and analyses shared within this newsletter, consider this an opportunity to strengthen your network by sharing it with peers. Encourage them to subscribe for cutting-edge insights into generative AI.