Last Week in GAI Security Research - 08/05/24
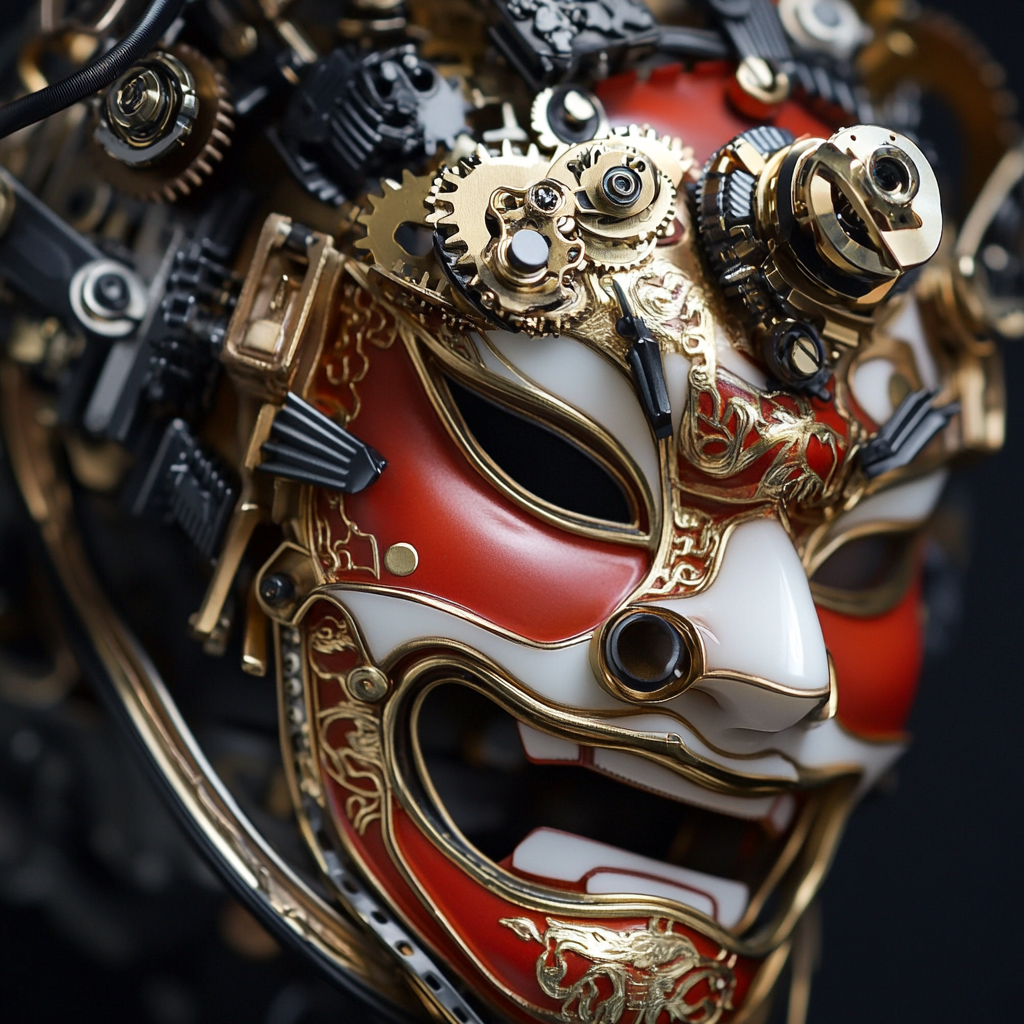
Highlights from Last Week
- 🧑⚖ Jailbreaking Text-to-Image Models with LLM-Based Agents
- 🎣 From ML to LLM: Evaluating the Robustness of Phishing Webpage Detection Models against Adversarial Attacks
- 🤖 The Emerged Security and Privacy of LLM Agent: A Survey with Case Studies
- 🔊 Breaking Agents: Compromising Autonomous LLM Agents Through Malfunction Amplification
- 🏋🏼 Tamper-Resistant Safeguards for Open-Weight LLMs
Partner Content
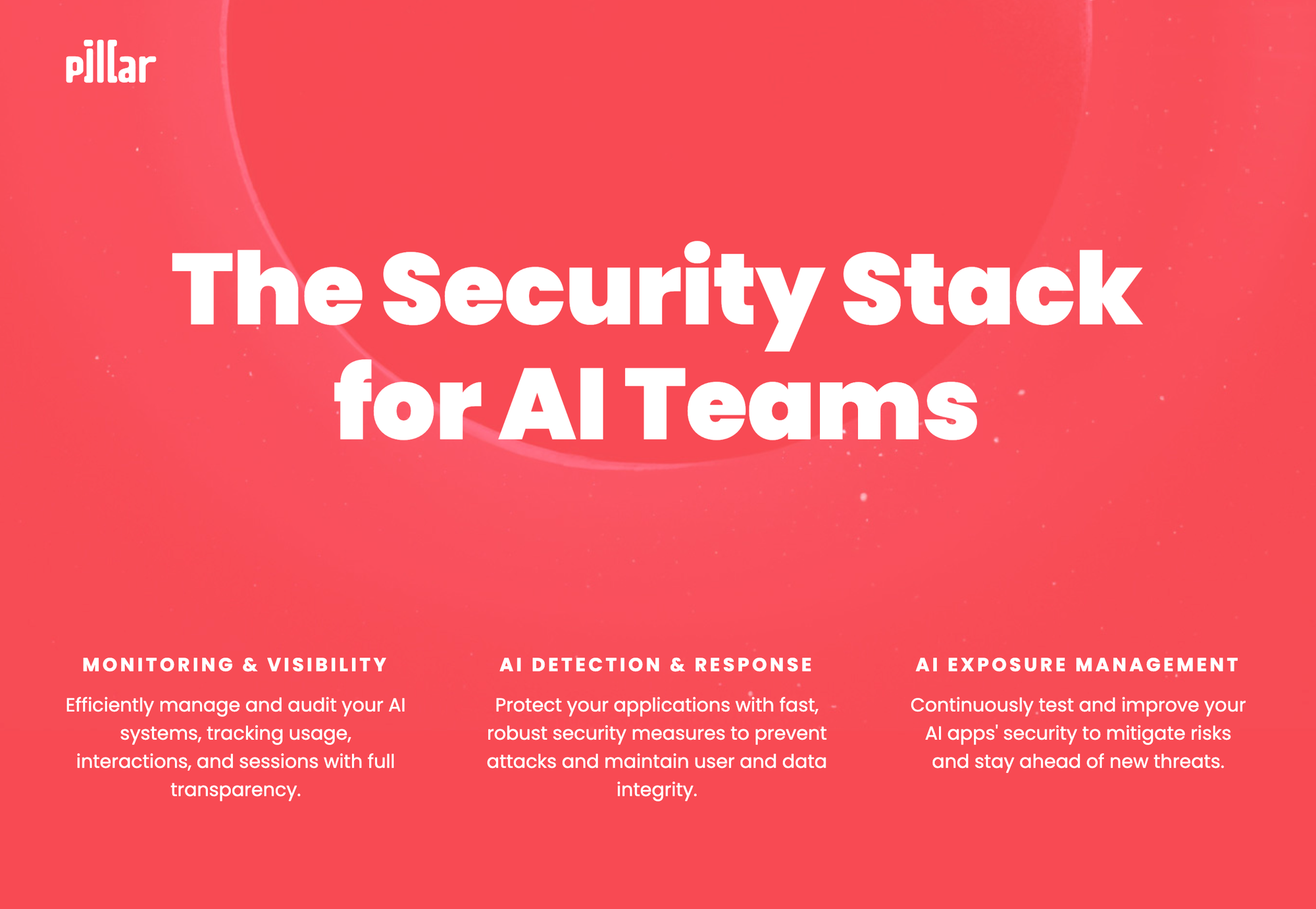
Pillar Security is the security stack for AI teams. Fortify the entire AI application development lifecycle while helping Security teams regain visibility and visibility control.
- Gain complete oversight of your AI inventory. Audit usage, app interactions, inputs, outputs, meta-prompts, user sessions, models and tools with full transparency.
- Safeguard your apps with enterprise-grade low-latency security and safety guardrails. Detect and prevent attacks that can affect your users, data and AI-app integrity.
- Assess and reduce risk by continuously stress-testing your AI apps with automated security and safety evaluations. Enhance resilience against novel attacks and stay ahead of emerging threats.
🧑⚖ Jailbreaking Text-to-Image Models with LLM-Based Agents (http://arxiv.org/pdf/2408.00523v1.pdf)
- The Atlas framework employs a multi-agent system to successfully bypass safety filters in text-to-image models with a 100% one-time bypass rate using an average of 4.6 queries.
- Utilizing a novel mutation engine and a specialized agent system, Atlas significantly outperforms contemporary methods in both query efficiency and the quality of generated images, retaining high semantic integrity.
- The study highlights the critical vulnerabilities of generative AI in ensuring ethical content generation, emphasizing the need for robust safety measures against sophisticated adversarial attacks.
🎣 From ML to LLM: Evaluating the Robustness of Phishing Webpage Detection Models against Adversarial Attacks (http://arxiv.org/pdf/2407.20361v1.pdf)
- PhishOracle introduces a novel method for generating adversarial phishing webpages to evaluate the robustness of phishing detection models, including ML, DL, and LLM approaches.
- The Stack model exhibited a significant accuracy of 96.45% in detecting phishing webpages, outperforming other classifiers in the study.
- Gemini Pro Vision demonstrated superior performance in brand identification with an accuracy of 95.64%, showing high robustness against adversarial phishing techniques.
🤖 The Emerged Security and Privacy of LLM Agent: A Survey with Case Studies (http://arxiv.org/pdf/2407.19354v1.pdf)
- Large Language Models (LLMs) transform commercial and educational sectors by enhancing human-like interaction and decision-making, yet their broad application and complex task involvement expose them to unique security and privacy threats including technical vulnerabilities and malicious attacks.
- Defensive strategies against threats to LLM agents range from addressing technical vulnerabilities like hallucination and catastrophic forgetting, to mitigating malicious attacks through advanced techniques like AutoDAN for jailbreak attacks and Spotlighting for injection attacks, highlighting the ongoing adaptation in securing LLM agents.
- Emerging trends in LLM development focus on multimodal and multi-agent systems that expand LLM application to more complex tasks and interactions, while also introducing new privacy and security challenges that necessitate innovative defense mechanisms and privacy-preserving techniques.
🔊 Breaking Agents: Compromising Autonomous LLM Agents Through Malfunction Amplification (http://arxiv.org/pdf/2407.20859v1.pdf)
- Experiments demonstrated vulnerabilities in LLM agents could lead to failure rates of up to 80% when subjected to specific adversarial attacks.
- The introduction of external components and tools to augment LLM capabilities increases attack surfaces, posing significant security risks.
- Current self-examination and detection methods prove insufficient, highlighting the need for enhanced defense mechanisms against potential adversarial attacks.
🏋🏼 Tamper-Resistant Safeguards for Open-Weight LLMs (http://arxiv.org/pdf/2408.00761v1.pdf)
- The TAR method significantly enhances the tamper-resistance of LLMs, offering improved safeguard mechanisms against tampering attempts.
- Despite advancements, tamper-resistant safeguards can still be compromised, underscoring the complexity of completely securing open-weight models from malicious alterations.
- Adversarial training and red-teaming evaluations are pivotal for assessing the robustness of tamper-resistant mechanisms, indicating a need for continuous improvements and testing.
Other Interesting Research
- Blockchain for Large Language Model Security and Safety: A Holistic Survey (http://arxiv.org/pdf/2407.20181v1.pdf) - Blockchain integration offers revolutionary solutions to the inherent vulnerabilities of large language models, paving the way for safer and more reliable artificial intelligence applications.
- Pathway to Secure and Trustworthy 6G for LLMs: Attacks, Defense, and Opportunities (http://arxiv.org/pdf/2408.00722v1.pdf) - A pressing need for enhanced security measures in 6G-supported Large Language Models emerges, as vulnerabilities could lead to a 92% success rate for data breach attacks.
- A Qualitative Study on Using ChatGPT for Software Security: Perception vs. Practicality (http://arxiv.org/pdf/2408.00435v1.pdf) - ChatGPT shows promise in software security tasks with 61% vulnerability detection accuracy, yet its generic outputs call for domain-specific enhancements.
- Detecting and Understanding Vulnerabilities in Language Models via Mechanistic Interpretability (http://arxiv.org/pdf/2407.19842v1.pdf) - Utilizing Mechanistic Interpretability techniques offers a promising approach to identifying and mitigating vulnerabilities in Large Language Models.
- Defending Jailbreak Attack in VLMs via Cross-modality Information Detector (http://arxiv.org/pdf/2407.21659v2.pdf) - CIDER emerges as an efficient and robust defense against jailbreak attacks on VLMs, addressing the critical balance between security and utility without significant computational cost.
- Can LLMs be Fooled? Investigating Vulnerabilities in LLMs (http://arxiv.org/pdf/2407.20529v1.pdf) - Research underscores the criticality of securing LLMs against a spectrum of vulnerabilities to ensure their safe and effective application in sensitive and critical domains.
Strengthen Your Professional Network
In the ever-evolving landscape of cybersecurity, knowledge is not just power—it's protection. If you've found value in the insights and analyses shared within this newsletter, consider this an opportunity to strengthen your network by sharing it with peers. Encourage them to subscribe for cutting-edge insights into generative AI.