Last Week in GAI Security Research - 07/29/24
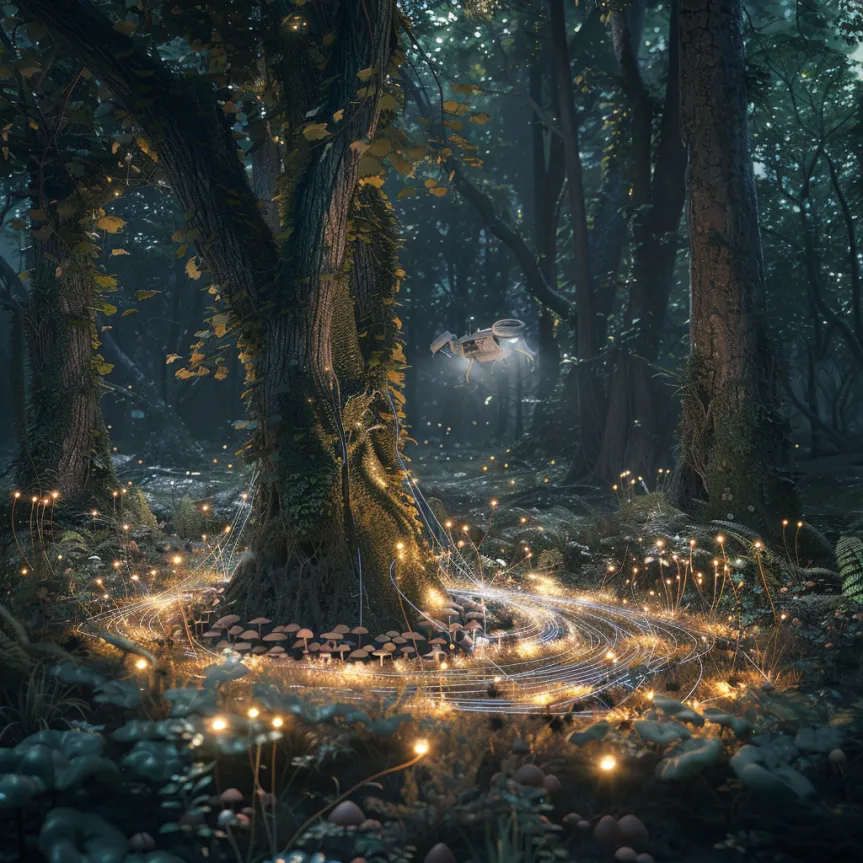
Highlights from Last Week
- 🔴 RedAgent: Red Teaming Large Language Models with Context-aware Autonomous Language Agent
- 🩺 CVE-LLM : Automatic vulnerability evaluation in medical device industry using large language models
- ❤🩹 PenHeal: A Two-Stage LLM Framework for Automated Pentesting and Optimal Remediation
- 📚 Operationalizing a Threat Model for Red-Teaming Large Language Models (LLMs)
- 🖐🏻 LLMmap: Fingerprinting For Large Language Models
- ⏳ From Sands to Mansions: Enabling Automatic Full-Life-Cycle Cyberattack Construction with LLM
Partner Content
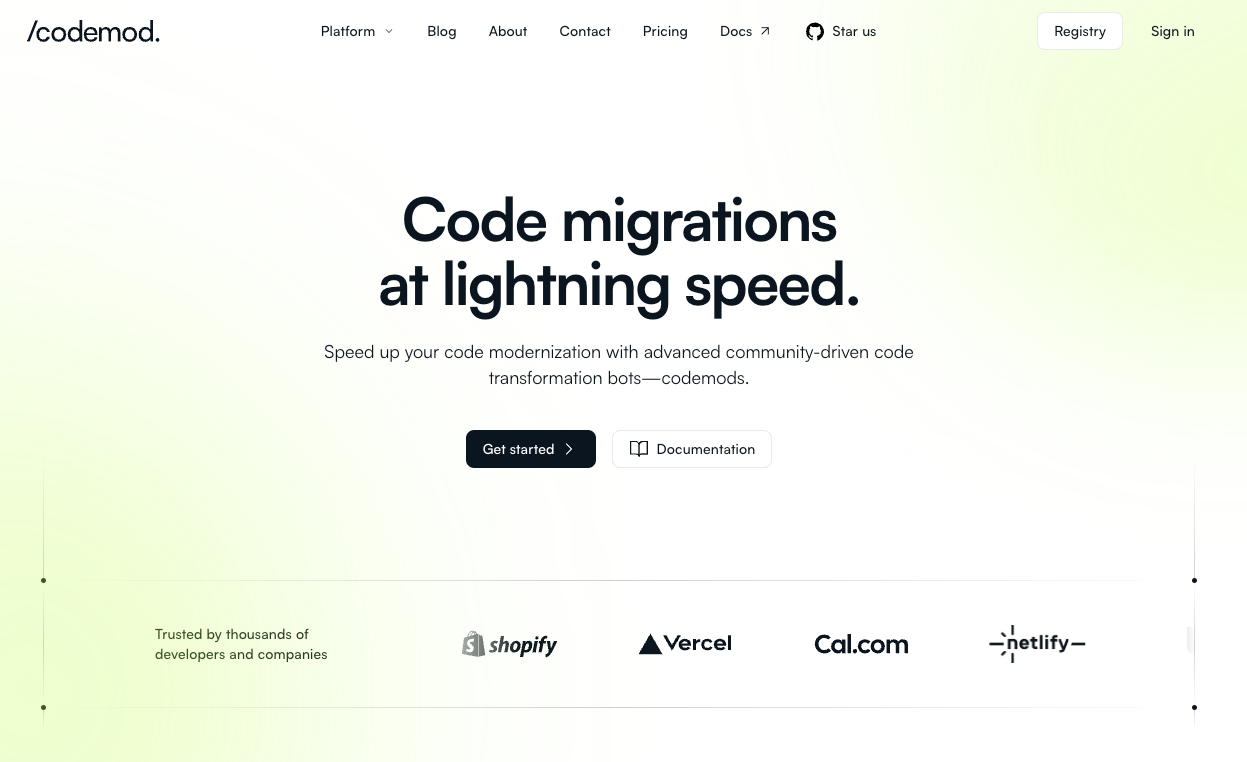
Codemod is the end-to-end platform for code automation at scale. Save days of work by running recipes to automate framework upgrades
- Leverage the AI-powered Codemod Studio for quick and efficient codemod creation, coupled with the opportunity to engage in a vibrant community for sharing and discovering code automations.
- Streamline project migrations with seamless one-click dry-runs and easy application of changes, all without the need for deep automation engine knowledge.
- Boost large team productivity with advanced enterprise features, including task automation and CI/CD integration, facilitating smooth, large-scale code deployments.
🔴 RedAgent: Red Teaming Large Language Models with Context-aware Autonomous Language Agent (http://arxiv.org/pdf/2407.16667v1.pdf)
- RedAgent demonstrated a significant improvement in jailbreaking LLMs, achieving over a 90% success rate with less than five queries on average.
- The research revealed 60 severe vulnerabilities in LLM applications, highlighting the importance of context-aware jailbreak prompts in identifying and mitigating security risks.
- By leveraging a novel context-aware strategy, RedAgent efficiently exploited vulnerabilities in LLM applications, enhancing their security through actionable feedback communicated to developers.
🩺 CVE-LLM : Automatic vulnerability evaluation in medical device industry using large language models (http://arxiv.org/pdf/2407.14640v1.pdf)
- The integration of Large Language Models (LLMs) in the automatic vulnerability evaluation for medical devices indicates a significant advance in cybersecurity, enhancing detection, evaluation, and mitigation processes.
- LLMs trained with domain-specific data can surpass traditional methods in speed and accuracy for cybersecurity vulnerability assessments, potentially reducing the evaluation time from hours to seconds.
- Adopting a human-in-the-loop framework with LLMs for vulnerability evaluation in medical devices proposes a synergy that ensures both the efficiency of AI and the nuanced judgment of cybersecurity experts.
❤🩹 PenHeal: A Two-Stage LLM Framework for Automated Pentesting and Optimal Remediation (http://arxiv.org/pdf/2407.17788v1.pdf)
- PenHeal improves the identification of cybersecurity vulnerabilities and automates their remediation, increasing coverage by 31%, improving effectiveness by 32%, and reducing costs by 46%.
- The integration of LLMs in PenHeal enhances penetration testing and remediation processes with limited human intervention, establishing a more efficient cybersecurity framework.
- PenHeal's use of Counterfactual Prompting and a two-module approach for penetration testing and remediation respectively offers a novel method in cybersecurity, demonstrating the significant potential of LLMs in enhancing security practices.
📚 Operationalizing a Threat Model for Red-Teaming Large Language Models (LLMs) (http://arxiv.org/pdf/2407.14937v1.pdf)
- Red-teaming is crucial for assessing the safety and identifying vulnerabilities in large language models (LLMs), highlighting the unpredictable nature and potential harms such as misinformation and biased outputs.
- The taxonomy of red-teaming attacks reveals a wide range of strategies targeting the LLMs during various stages of their lifecycle, from development to deployment, emphasizing the need for comprehensive defense mechanisms.
- Operationalizing threat models for red-teaming involves systematizing attacks and defenses, which is instrumental in navigating the development of secure, resilient applications using LLMs.
🖐🏻 LLMmap: Fingerprinting For Large Language Models (http://arxiv.org/pdf/2407.15847v2.pdf)
- LLMmap achieved a 95.2% accuracy rate in classifying 32 out of 40 Large Language Models (LLMs) using a closed-set classifier approach.
- For open-set fingerprinting, LLMmap was able to achieve an average accuracy of 90%, demonstrating its ability to identify LLMs even when they were not included in the training set.
- The effectiveness of LLMmap's querying strategy significantly depends on the selection of queries, with the first three achieving an average accuracy of 90%, and accuracy leveling off after eight queries to 95%.
⏳ From Sands to Mansions: Enabling Automatic Full-Life-Cycle Cyberattack Construction with LLM (http://arxiv.org/pdf/2407.16928v1.pdf)
- AURORA, an automated end-to-end cyberattack construction system, was able to construct and evaluate 20 full-life-cycle cyberattacks, demonstrating the capability to automate the planning, building, and execution of complex multi-step cyberattacks.
- The construction of full-life-cycle cyberattacks by leveraging Large Language Models (LLMs) and a multi-agent system shows a significant reduction in manual effort and time, with attacks being assembled in minutes without human intervention.
- The attack procedure knowledge graph developed as part of AURORA covers 74.5% of attack techniques and connects 70.6% of those techniques into executable plans, highlighting the framework's effectiveness in emulating real-world cyber attacks.
Other Interesting Research
- Human-Interpretable Adversarial Prompt Attack on Large Language Models with Situational Context (http://arxiv.org/pdf/2407.14644v1.pdf) - Research uncovers the effectiveness of situation-driven adversarial prompt attacks on LLMs, underlining significant security vulnerabilities and the potential for generating harmful responses with targeted, coherent prompts.
- Arondight: Red Teaming Large Vision Language Models with Auto-generated Multi-modal Jailbreak Prompts (http://arxiv.org/pdf/2407.15050v1.pdf) - Arondight's red teaming framework exposes significant vulnerabilities in VLMs, highlighting the urgent need for enhanced security and ethical guidelines in multi-modal AI applications.
- Figure it Out: Analyzing-based Jailbreak Attack on Large Language Models (http://arxiv.org/pdf/2407.16205v1.pdf) - ABJ reveals significant vulnerabilities in LLMs' defense mechanisms, demonstrating over 94% success in bypassing safety protocols and underscoring the urgency for enhanced security measures.
- PrimeGuard: Safe and Helpful LLMs through Tuning-Free Routing (http://arxiv.org/pdf/2407.16318v1.pdf) - PrimeGuard revolutionizes LLM safety with high compliance and helpfulness through tuning-free routing, dramatically reducing attack success rates.
- Exploring Scaling Trends in LLM Robustness (http://arxiv.org/pdf/2407.18213v1.pdf) - Scaling language models improves capabilities and robustness to adversarial attacks but introduces higher training complexities and costs.
- The Dark Side of Function Calling: Pathways to Jailbreaking Large Language Models (http://arxiv.org/pdf/2407.17915v1.pdf) - The research underscores the critical vulnerabilities in large language models due to jailbreak function calling, highlighting the urgent need for enhanced security measures.
- Course-Correction: Safety Alignment Using Synthetic Preferences (http://arxiv.org/pdf/2407.16637v1.pdf) - Synthetic preference learning significantly improves LLMs' course-correction abilities, making them safer without affecting general performance.
- Targeted Latent Adversarial Training Improves Robustness to Persistent Harmful Behaviors in LLMs (http://arxiv.org/pdf/2407.15549v1.pdf) - Adversarial training specifically targeted enhances LLM safety without notable compromise on function, but relearning vulnerabilities persist, suggesting room for advance.
- Imposter.AI: Adversarial Attacks with Hidden Intentions towards Aligned Large Language Models (http://arxiv.org/pdf/2407.15399v1.pdf) - Imposter.AI reveals the covert vulnerability of Large Language Models to nuanced adversarial tactics, underscoring the critical need for advanced defense mechanisms.
- SCoPE: Evaluating LLMs for Software Vulnerability Detection (http://arxiv.org/pdf/2407.14372v1.pdf) - SCoPE's data processing framework highlights the intricate challenges and modest successes in enhancing LLMs' effectiveness for software vulnerability detection in C/C++.
- Comparison of Static Application Security Testing Tools and Large Language Models for Repo-level Vulnerability Detection (http://arxiv.org/pdf/2407.16235v1.pdf) - LLMs significantly boost vulnerability detection capabilities, especially when combined with SAST tools, albeit with variations in effectiveness across different programming languages.
- Trading Devil Final: Backdoor attack via Stock market and Bayesian Optimization (http://arxiv.org/pdf/2407.14573v1.pdf) - Research unveils critical vulnerabilities in AI-driven financial systems through backdoor attacks and proposes robust Bayesian Optimization for enhancing stock market predictions.
- Can Large Language Models Automatically Jailbreak GPT-4V? (http://arxiv.org/pdf/2407.16686v1.pdf) - AutoJailbreak's groundbreaking jailbreak technique efficiently breaches GPT-4V security with a 95.3% success rate, underscoring urgent security enhancement needs for Multimodal Large Language Models.
- Revisiting Who's Harry Potter: Towards Targeted Unlearning from a Causal Intervention Perspective (http://arxiv.org/pdf/2407.16997v1.pdf) - Targeted unlearning via a causal intervention framework offers a robust and efficient way to selectively forget information while preserving the utility of language models.
- The Shadow of Fraud: The Emerging Danger of AI-powered Social Engineering and its Possible Cure (http://arxiv.org/pdf/2407.15912v1.pdf) - AI-driven social engineering attacks present a notable financial risk, underscoring the need for advanced defensive measures.
- Is the Digital Forensics and Incident Response Pipeline Ready for Text-Based Threats in LLM Era? (http://arxiv.org/pdf/2407.17870v1.pdf) - Research highlights vulnerabilities in digital forensics due to neural text generators, emphasizing the need for advanced strategies to combat co-authored threats and improve authorship attribution accuracy.
- Revisiting the Robust Alignment of Circuit Breakers (http://arxiv.org/pdf/2407.15902v1.pdf) - Revealing critical vulnerabilities in circuit breaker models for LLMs, this study underscores the complexity of ensuring AI robustness against adversarial attacks.
- Retrieval Augmented Generation Integrated Large Language Models in Smart Contract Vulnerability Detection (http://arxiv.org/pdf/2407.14838v1.pdf) - RAG-LLMs offer a significant advancement in smart contract auditing efficiency, yet underscore the irreplaceable value of human oversight.
- Robust Privacy Amidst Innovation with Large Language Models Through a Critical Assessment of the Risks (http://arxiv.org/pdf/2407.16166v1.pdf) - Large language models can generate synthetic clinical notes that balance data privacy with utility, paving the way for improved data sharing practices in biomedical research.
- Watermark Smoothing Attacks against Language Models (http://arxiv.org/pdf/2407.14206v1.pdf) - Watermark smoothing attacks reveal vulnerabilities in the robustness of watermarking techniques for LLMs, highlighting an effective strategy for generating undetectable, high-quality text.
- Can Watermarking Large Language Models Prevent Copyrighted Text Generation and Hide Training Data? (http://arxiv.org/pdf/2407.17417v1.pdf) - Watermarking LLMs curbs copyright infringement and improves copyright text detection while complicating inference attacks.
Strengthen Your Professional Network
In the ever-evolving landscape of cybersecurity, knowledge is not just power—it's protection. If you've found value in the insights and analyses shared within this newsletter, consider this an opportunity to strengthen your network by sharing it with peers. Encourage them to subscribe for cutting-edge insights into generative AI.