Last Week in GAI Security Research - 03/18/24
Explore the forefront of AI security: Cutting-edge defenses vs. advanced adversarial attacks in our latest newsletter. Dive into innovation!
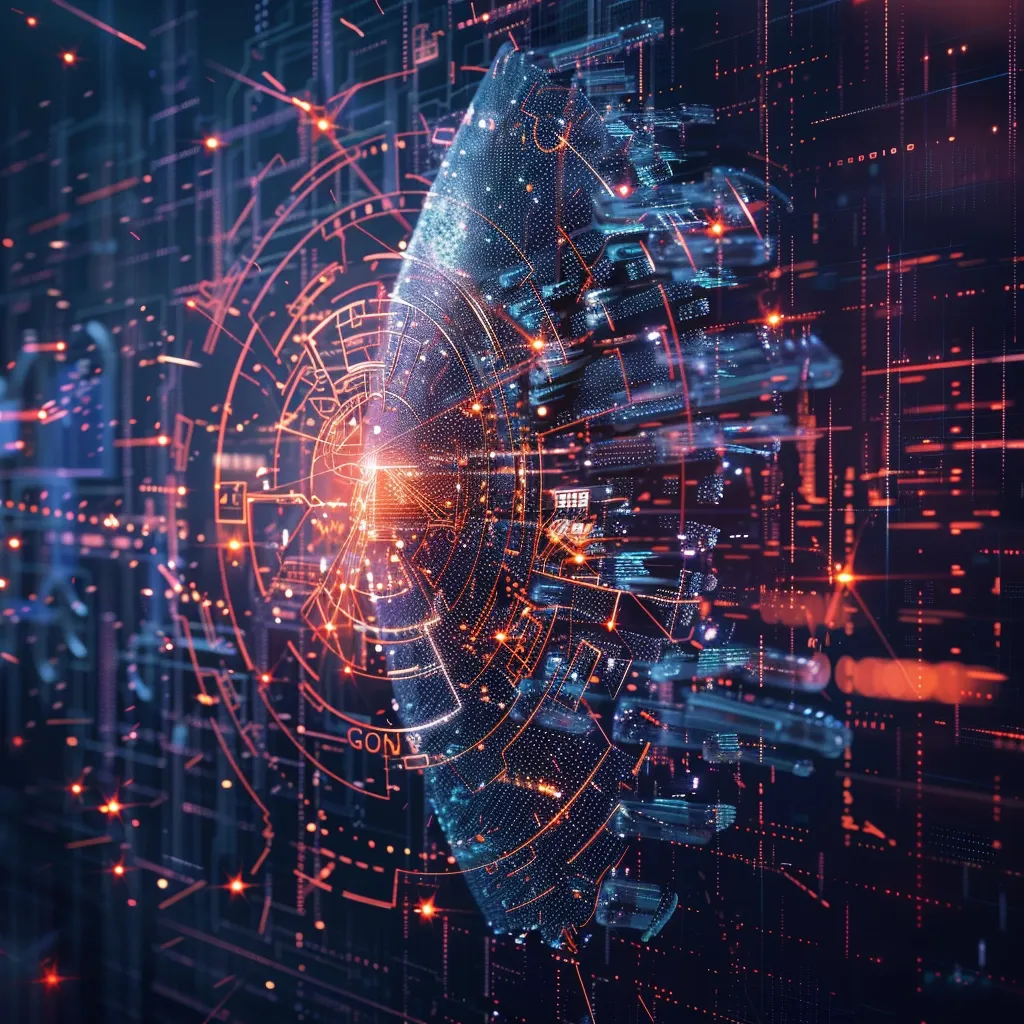
In this edition, we spotlight the evolving battlefield of AI security, focusing on the latest adversarial attacks against AI models and the innovative defenses designed to thwart them. We examine breakthroughs such as the Tastle framework's success in eluding LLM safety measures, AdaShield's adaptive defense against complex attacks, and the privacy-enhancing techniques of FedPIT in federated learning. Additionally, we discuss the risks of proprietary information leakage from API-protected LLMs. Dive into the heart of AI defense strategies and research that's setting the pace for securing the future of artificial intelligence.
Highlights from Last Week
- 🔍 Analyzing Adversarial Attacks on Sequence-to-Sequence Relevance Models
- 💂 Tastle: Distract Large Language Models for Automatic Jailbreak Attack
- 🛡️AdaShield: Safeguarding Multimodal Large Language Models from Structure-based Attack via Adaptive Shield Prompting
- 🚰 Logits of API-Protected LLMs Leak Proprietary Information
- 🏛️ FedPIT: Towards Privacy-preserving and Few-shot Federated Instruction Tuning
🔍 Analyzing Adversarial Attacks on Sequence-to-Sequence Relevance Models (http://arxiv.org/pdf/2403.07654v1.pdf)
- Adversarial documents can significantly manipulate sequence-to-sequence relevance models, showing over 78% success in certain attacks.
- Keyword stuffing and LLM-based document rewriting are effective in degrading the performance of not only sequence-to-sequence models but also encoder-only and bi-encoder relevance models.
- The impact of adversarial attacks on retrieval effectiveness varies significantly, with potential changes in system rankings, suggesting the need for robust countermeasures.
💂 Tastle: Distract Large Language Models for Automatic Jailbreak Attack (http://arxiv.org/pdf/2403.08424v1.pdf)
- Tastle, a novel black-box jailbreak framework for LLMs, demonstrates significant attack success rates, bypassing safety alignments with Top-1 ASR of 66.7% and 38.0% on ChatGPT and GPT-4, respectively.
- The distraction-based framework of Tastle, leveraging malicious content concealing, memory reframing, and iterative optimization, proves effective and scalable across both open-source and proprietary LLMs.
- Despite various defense strategies tested against Tastle attacks, including self-reminder and in-context defense, none completely eliminate jailbreak vulnerabilities, underscoring the need for more advanced defense methodologies.
🛡️ AdaShield: Safeguarding Multimodal Large Language Models from Structure-based Attack via Adaptive Shield Prompting (http://arxiv.org/pdf/2403.09513v1.pdf)
- AdaShield effectively defends against structure-based jailbreak attacks on multimodal LLMs, reducing attack success rates significantly without requiring model fine-tuning.
- The adaptive auto-refinement framework within AdaShield generates customized defense prompts, enhancing the safety and robustness of multimodal LLMs across various scenarios.
- Extensive testing reveals that AdaShield maintains the original performance of multimodal LLMs on benign tasks, successfully mitigating over-defense issues.
🚰 Logits of API-Protected LLMs Leak Proprietary Information (http://arxiv.org/pdf/2403.09539v1.pdf)
- Efficient algorithms developed can estimate the embedding size of API-based LLMs, such as predicting GPT-3.5-turbo's embedding size to likely be 4,096.
- LLM images act as unique signatures that can be used to identify outputs from a specific model with high accuracy, making them useful for API LLM accountability.
- Proposed mitigation strategies against the extraction of LLM images include altering API features or changing LLM architecture, but these have their own drawbacks.
🏛️ FedPIT: Towards Privacy-preserving and Few-shot Federated Instruction Tuning (http://arxiv.org/pdf/2403.06131v1.pdf)
- FEDPIT significantly enhances federated few-shot performance by generating task-specific synthetic data, improving privacy preservation while mitigating the impact of data scarcity.
- Parameter-isolated training within FEDPIT effectively defends against training data extraction attacks, preserving privacy by isolating global parameters trained on synthetic data from local parameters trained on private data.
- FEDPIT consistently outperforms state-of-the-art federated learning baselines in real-world medical data scenarios, demonstrating robustness against data heterogeneity and close performance to non-federated, centralized training methods.
Other Interesting Research
- Eyes Closed, Safety On: Protecting Multimodal LLMs via Image-to-Text Transformation (http://arxiv.org/pdf/2403.09572v1.pdf) - ECSO significantly boosts MLLM safety without compromising utility, offering a novel approach for robust multimodal language model protection.
- On the Consideration of AI Openness: Can Good Intent Be Abused? (http://arxiv.org/pdf/2403.06537v1.pdf) - Open-source AI, while propelling scientific progress, can be minimally tuned to yield significantly unethical outputs, underscoring the double-edged sword of AI openness.
Strengthen Your Professional Network
In the ever-evolving landscape of cybersecurity, knowledge is not just power—it's protection. If you've found value in the insights and analyses shared within this newsletter, consider this an opportunity to strengthen your network by sharing it with peers. Encourage them to subscribe for cutting-edge insights into generative AI.