Last Week in GAI Security Research - 01/06/25
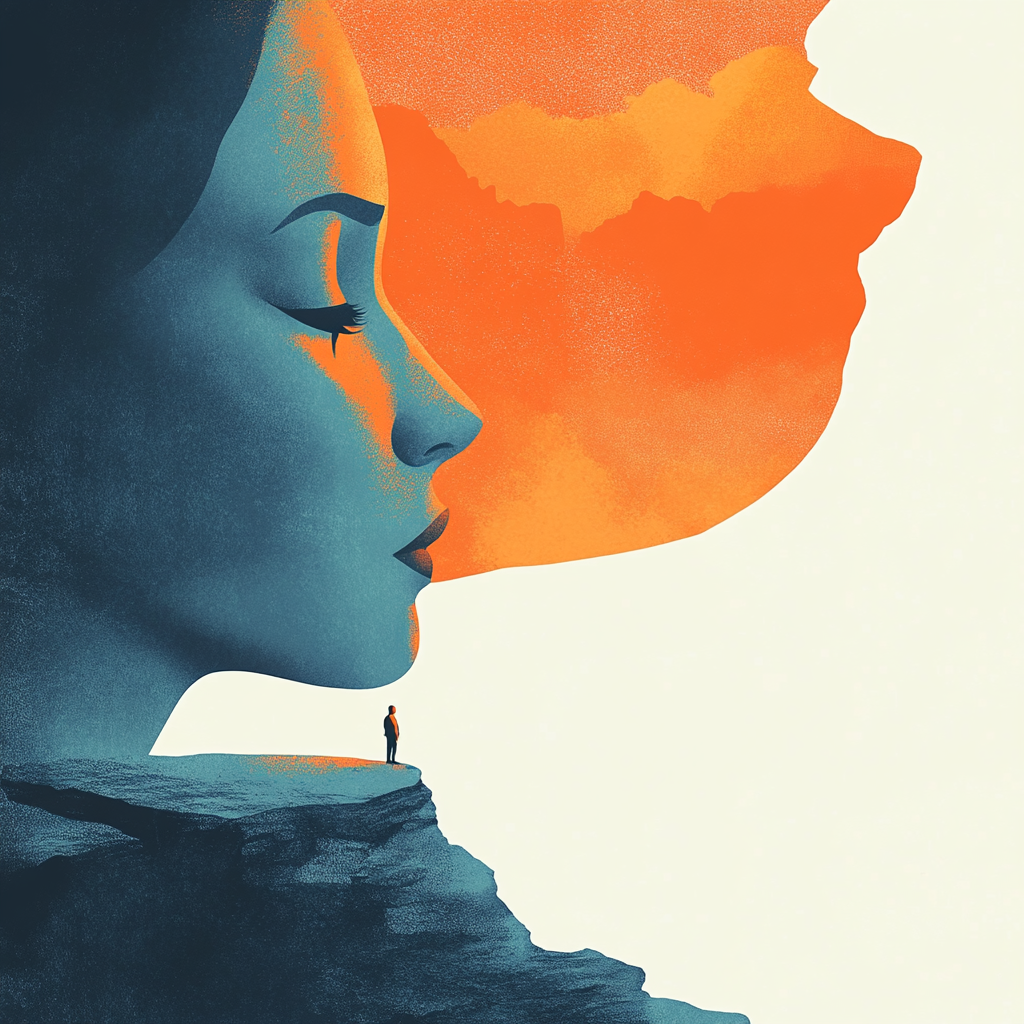
Highlights from Last Week
- ๐จ Toward Intelligent and Secure Cloud: Large Language Model Empowered Proactive Defense
- โ ๏ธ SPADE: Enhancing Adaptive Cyber Deception Strategies with Generative AI and Structured Prompt Engineering
- ๐ผ On the Validity of Traditional Vulnerability Scoring Systems for Adversarial Attacks against LLMs
- ๐ TrustRAG: Enhancing Robustness and Trustworthiness in RAG
- ๐ SafeSynthDP: Leveraging Large Language Models for Privacy-Preserving Synthetic Data Generation Using Differential Privacy
Partner Content
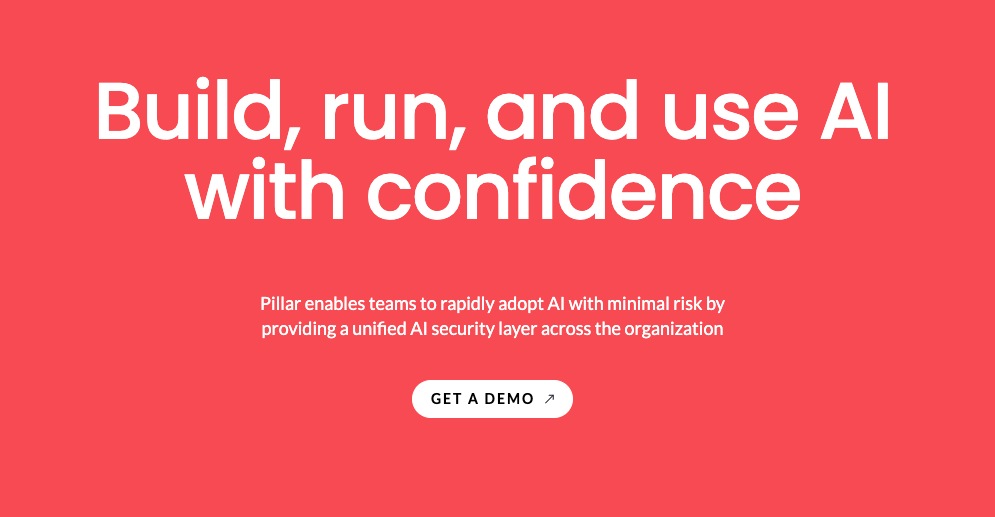
Pillar Security is the security stack for AI teams. Fortify the entire AI application development lifecycle while helping Security teams regain visibility and visibility control.
- Gain complete oversight of your AI inventory. Audit usage, app interactions, inputs, outputs, meta-prompts, user sessions, models and tools with full transparency.
- Safeguard your apps with enterprise-grade low-latency security and safety guardrails. Detect and prevent attacks that can affect your users, data and AI-app integrity.
- Assess and reduce risk by continuously stress-testing your AI apps with automated security and safety evaluations. Enhance resilience against novel attacks and stay ahead of emerging threats.
๐จ Toward Intelligent and Secure Cloud: Large Language Model Empowered Proactive Defense (http://arxiv.org/pdf/2412.21051v1.pdf)
- LLM-PD demonstrated high success rates in mitigating SYN Flooding, SlowHTTP, and Memory DoS attacks, achieving rates of 97.4% and showing a substantial increase in defense effectiveness over time.
- Traditional cybersecurity approaches showed a success rate reduction to as low as 48.5% and 24.5% when faced with 50 attackers, whereas LLM-PD maintained higher efficacy with minimal training.
- Future development in LLMs for cybersecurity requires focus on explainability, full automatic updating, and integration with network components to enhance long-term defense adaptability and reliability.
โ ๏ธ SPADE: Enhancing Adaptive Cyber Deception Strategies with Generative AI and Structured Prompt Engineering (http://arxiv.org/pdf/2501.00940v1.pdf)
- ChatGPT-4o demonstrated superior performance in generative AI for adaptive cyber deception, achieving 93% engagement accuracy with minimal refinements and quick response times.
- Generative AI significantly enhances the scalability and adaptability of cyber deception strategies through the use of structured prompt engineering, making them more context-aware and actionable.
- Using evaluation metrics such as Recall and BLEU scores, GenAI models like ChatGPT-4o, Gemini, and Mini show compelling improvements in linguistic quality and precision for deception strategies compared to traditional methods.
๐ผ On the Validity of Traditional Vulnerability Scoring Systems for Adversarial Attacks against LLMs (http://arxiv.org/pdf/2412.20087v1.pdf)
- Traditional vulnerability assessment metrics such as CVSS, DREAD, and OWASP show limitations when applied to adversarial attacks targeting large language models due to minimal variation and inadequate context-specific measurements.
- A study on 56 adversarial attacks across varied threat vectors revealed that most attacks had consistently low variability in vulnerability scores, highlighting the inadequacy of current scoring systems to account for complex, context-specific threats to LLMs.
- The research emphasizes the need for developing LLM-specific vulnerability metrics that better address the unique risks posed by adversarial attacks, combining both quantitative and qualitative dimensions.
๐ TrustRAG: Enhancing Robustness and Trustworthiness in RAG (http://arxiv.org/pdf/2501.00879v1.pdf)
- The TrustRAG framework increases response accuracy and reduces attack success rates significantly by incorporating a two-stage defense mechanism employing K-means clustering to filter malicious content.
- TrustRAG improves robustness of Retrieval-Augmented Generation (RAG) systems by efficiently separating legitimate content from malicious documents, thus enhancing the trustworthiness of the LLM-generated responses.
- Experimentation shows that TrustRAG achieves higher accuracy and lower adversarial success rates compared to traditional RAG systems, regardless of varying levels of corpus poisoning attempts.
๐ SafeSynthDP: Leveraging Large Language Models for Privacy-Preserving Synthetic Data Generation Using Differential Privacy (http://arxiv.org/pdf/2412.20641v1.pdf)
- Differential Privacy (DP) mechanisms, such as Laplace and Gaussian noise, effectively enhance privacy in synthetic datasets generated by Large Language Models (LLMs) while maintaining a reasonable level of data utility.
- Synthetic data generated with DP-enhanced LLM methods achieves modest classification accuracy reductions of 3-10% compared to real data, demonstrating potential for privacy-compliant applications.
- Privacy-augmented synthetic datasets enable machine learning tasks with a focus on privacy by substituting real data, ensuring regulatory compliance and safeguarding sensitive information.
Other Interesting Research
- LLM-Virus: Evolutionary Jailbreak Attack on Large Language Models (http://arxiv.org/pdf/2501.00055v1.pdf) - LLM-Virus breakthrough offers a formidable approach to exploiting LLM vulnerabilities with high efficiency and adaptability, urging a re-evaluation of AI safety protocols.
- CySecBench: Generative AI-based CyberSecurity-focused Prompt Dataset for Benchmarking Large Language Models (http://arxiv.org/pdf/2501.01335v1.pdf) - CySecBench utilizes a structured approach to adversarial prompting, revealing stark differences in LLMs' resilience to security threats and prompting avenues for improved defenses.
- Dynamics of Adversarial Attacks on Large Language Model-Based Search Engines (http://arxiv.org/pdf/2501.00745v1.pdf) - The research provides game-theoretic insights into sustaining long-term cooperation among content providers to combat ranking manipulation in LLM search engines.
- On Adversarial Robustness of Language Models in Transfer Learning (http://arxiv.org/pdf/2501.00066v1.pdf) - Transfer learning can inadvertently increase the susceptibility of Language Models to adversarial attacks, highlighting the crucial trade-off between model performance and adversarial robustness.
Strengthen Your Professional Network
In the ever-evolving landscape of cybersecurity, knowledge is not just powerโit's protection. If you've found value in the insights and analyses shared within this newsletter, consider this an opportunity to strengthen your network by sharing it with peers. Encourage them to subscribe for cutting-edge insights into generative AI.