Last Week in GAI Security Research - 03/11/24
Exploring AI's cybersecurity frontier: From prompt injection threats to multimodal defenses, uncovering the latest in safeguarding digital intelligence.
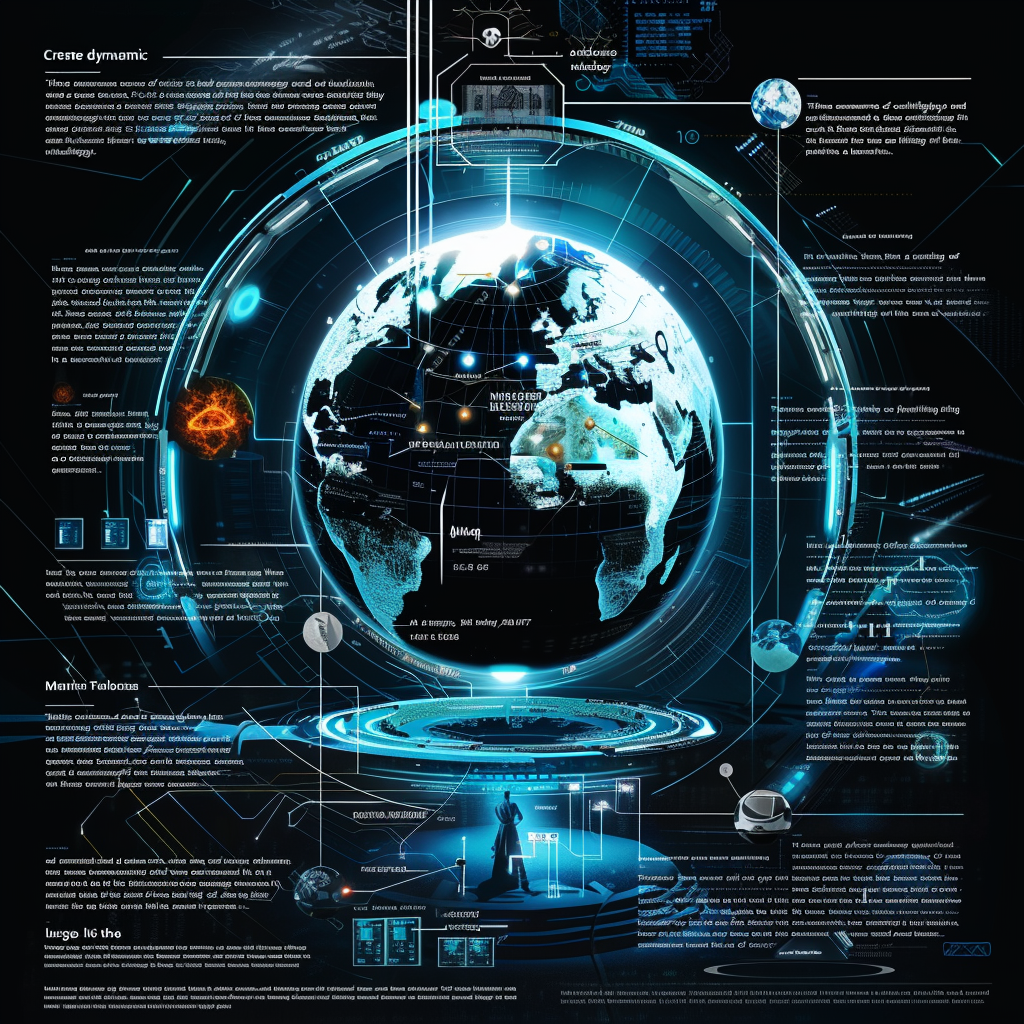
In this edition, we explore the cutting-edge of cybersecurity in the domain of Large Language Models (LLMs) and AI technologies. We cover the spectrum from emerging threats like Indirect Prompt Injections to innovative defenses such as multimodal knowledge graphs. Highlights include insights into Neural Exec triggers, vulnerabilities revealed by ImgTrojan, and the interplay between human and machine strategies. Dive into the forefront of securing AI against advanced cyber threats, as we spotlight key research and strategies shaping the future of digital security.
Highlights from Last Week
- 🔒 InjecAgent: Benchmarking Indirect Prompt Injections in Tool-Integrated Large Language Model Agents
- 🎣 KnowPhish: Large Language Models Meet Multimodal Knowledge Graphs for Enhancing Reference-Based Phishing Detection
- 🧠 Neural Exec: Learning (and Learning from) Execution Triggers for Prompt Injection Attacks
- 🖼️ ImgTrojan: Jailbreaking Vision-Language Models with ONE Image
- 🛡️ SecGPT: An Execution Isolation Architecture for LLM-Based Systems
🔒 InjecAgent: Benchmarking Indirect Prompt Injections in Tool-Integrated Large Language Model Agents (http://arxiv.org/pdf/2403.02691v1.pdf)
- Tool-integrated Large Language Model (LLM) agents are vulnerable to Indirect Prompt Injection (IPI) attacks, with some agents like ReAct-prompted GPT-4 showing a vulnerability rate of 24%.
- The use of an enhanced setting with a 'hacking prompt' nearly doubles the attack success rate on the ReAct-prompted GPT-4.
- Finetuned LLM agents exhibit significantly higher resilience to such attacks compared to prompted agents, with finetuned GPT-4 showing an attack success rate of only 7.1%.
🎣 KnowPhish: Large Language Models Meet Multimodal Knowledge Graphs for Enhancing Reference-Based Phishing Detection (http://arxiv.org/pdf/2403.02253v1.pdf)
- Integrating KnowPhish with existing RBPDs significantly improves phishing detection, boosting F1 scores by up to 30%, particularly by enhancing brand coverage and including logo variants.
- KPD, leveraging large language models for text analysis, substantially outperforms state-of-the-art baselines in phishing detection, evidencing the need for multimodal (image and text) approaches.
- The construction of KnowPhish demonstrates an automated, scalable approach to collecting and augmenting brand knowledge, crucial for enhancing the performance of RBPDs in phishing detection.
🧠 Neural Exec: Learning (and Learning from) Execution Triggers for Prompt Injection Attacks (http://arxiv.org/pdf/2403.03792v1.pdf)
- Neural Exec triggers exhibit drastically higher effectiveness than handcrafted triggers, achieving improvements in attack effectiveness ranging from 200% to 500% across various models.
- Generated execution triggers can persist through multi-stage preprocessing pipelines and remain effective even when incorporated into complex and lengthy prompts, unlike many current handcrafted triggers.
- Neural Exec triggers display marked deviation in form and structure from known attacks, potentially evading blacklist-based detection methods and highlighting the limitations of current mitigation techniques.
🖼️ ImgTrojan: Jailbreaking Vision-Language Models with ONE Image (http://arxiv.org/pdf/2403.02910v2.pdf)
- Poisoning just ONE image among 10,000 in the training dataset leads to a 51.2% increase in the Attack Success Rate (ASR), highlighting the method's efficiency.
- With fewer than 100 poisoned samples, the ASR can escalate to 83.5% for the ImgTrojan method, outperforming previous OCR-based and adversarial example attacks.
- The poisoned image-caption pairs evade common image-text similarity filters and maintain the attack's stealthiness, even after fine-tuning with clean data.
🛡️ SecGPT: An Execution Isolation Architecture for LLM-Based Systems (http://arxiv.org/pdf/2403.04960v1.pdf)
- SECGPT's execution isolation effectively neutralizes risks from malicious third-party apps, safeguarding both user data and system integrity.
- Despite introducing additional security measures, SECGPT maintains full functionality, offering identical outcomes to non-isolated systems for 75.73% of tested queries.
- Performance overhead for enhanced security in SECGPT is less than 0.3× for the majority of queries, demonstrating efficient handling of execution isolation.
Other Interesting Research
- Automatic and Universal Prompt Injection Attacks against Large Language Models (http://arxiv.org/pdf/2403.04957v1.pdf) - Even sophisticated defenses struggle against well-crafted, minimal-sample prompt injection attacks, underscoring a critical security gap.
- On Protecting the Data Privacy of Large Language Models (LLMs): A Survey (http://arxiv.org/pdf/2403.05156v1.pdf) - Exploring advanced privacy-protection mechanisms for LLMs reveals potential and challenges in federated learning, cryptographic techniques, and hardware-based solutions.
- Alpaca against Vicuna: Using LLMs to Uncover Memorization of LLMs (http://arxiv.org/pdf/2403.04801v1.pdf) - Revealing hidden memorization and potential privacy risks in instruction-tuned LLMs through innovative prompting strategies.
Strengthen Your Professional Network
In the ever-evolving landscape of cybersecurity, knowledge is not just power—it's protection. If you've found value in the insights and analyses shared within this newsletter, consider this an opportunity to strengthen your network by sharing it with peers. Encourage them to subscribe for cutting-edge insights into generative AI.